注意
转到结尾 下载完整的示例代码。或者通过 JupyterLite 或 Binder 在浏览器中运行此示例
逻辑回归中的 L1 正则化和稀疏性#
当使用L1、L2和Elastic-Net惩罚项时,不同C值下解的稀疏度(零系数的百分比)比较。我们可以看到,较大的C值给予模型更大的自由度。相反,较小的C值对模型的约束更大。在L1惩罚项的情况下,这会导致更稀疏的解。正如预期的那样,Elastic-Net惩罚项的稀疏度介于L1和L2之间。
我们将8x8的数字图像分为两类:0-4和5-9。可视化显示了不同C值下模型的系数。
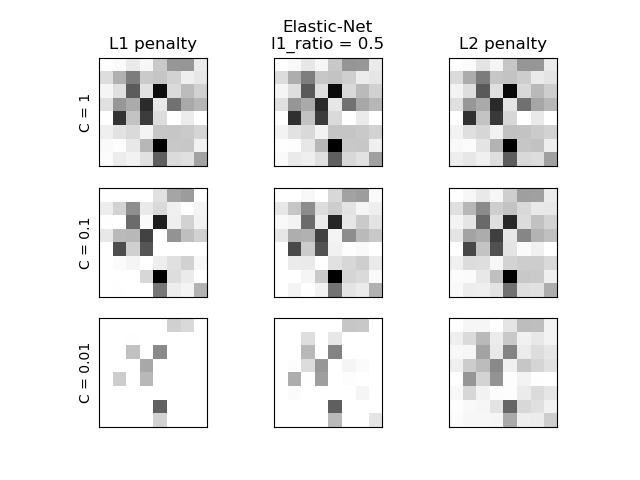
C=1.00
Sparsity with L1 penalty: 4.69%
Sparsity with Elastic-Net penalty: 4.69%
Sparsity with L2 penalty: 4.69%
Score with L1 penalty: 0.90
Score with Elastic-Net penalty: 0.90
Score with L2 penalty: 0.90
C=0.10
Sparsity with L1 penalty: 29.69%
Sparsity with Elastic-Net penalty: 14.06%
Sparsity with L2 penalty: 4.69%
Score with L1 penalty: 0.90
Score with Elastic-Net penalty: 0.90
Score with L2 penalty: 0.90
C=0.01
Sparsity with L1 penalty: 84.38%
Sparsity with Elastic-Net penalty: 68.75%
Sparsity with L2 penalty: 4.69%
Score with L1 penalty: 0.86
Score with Elastic-Net penalty: 0.88
Score with L2 penalty: 0.89
# Authors: The scikit-learn developers
# SPDX-License-Identifier: BSD-3-Clause
import matplotlib.pyplot as plt
import numpy as np
from sklearn import datasets
from sklearn.linear_model import LogisticRegression
from sklearn.preprocessing import StandardScaler
X, y = datasets.load_digits(return_X_y=True)
X = StandardScaler().fit_transform(X)
# classify small against large digits
y = (y > 4).astype(int)
l1_ratio = 0.5 # L1 weight in the Elastic-Net regularization
fig, axes = plt.subplots(3, 3)
# Set regularization parameter
for i, (C, axes_row) in enumerate(zip((1, 0.1, 0.01), axes)):
# Increase tolerance for short training time
clf_l1_LR = LogisticRegression(C=C, penalty="l1", tol=0.01, solver="saga")
clf_l2_LR = LogisticRegression(C=C, penalty="l2", tol=0.01, solver="saga")
clf_en_LR = LogisticRegression(
C=C, penalty="elasticnet", solver="saga", l1_ratio=l1_ratio, tol=0.01
)
clf_l1_LR.fit(X, y)
clf_l2_LR.fit(X, y)
clf_en_LR.fit(X, y)
coef_l1_LR = clf_l1_LR.coef_.ravel()
coef_l2_LR = clf_l2_LR.coef_.ravel()
coef_en_LR = clf_en_LR.coef_.ravel()
# coef_l1_LR contains zeros due to the
# L1 sparsity inducing norm
sparsity_l1_LR = np.mean(coef_l1_LR == 0) * 100
sparsity_l2_LR = np.mean(coef_l2_LR == 0) * 100
sparsity_en_LR = np.mean(coef_en_LR == 0) * 100
print(f"C={C:.2f}")
print(f"{'Sparsity with L1 penalty:':<40} {sparsity_l1_LR:.2f}%")
print(f"{'Sparsity with Elastic-Net penalty:':<40} {sparsity_en_LR:.2f}%")
print(f"{'Sparsity with L2 penalty:':<40} {sparsity_l2_LR:.2f}%")
print(f"{'Score with L1 penalty:':<40} {clf_l1_LR.score(X, y):.2f}")
print(f"{'Score with Elastic-Net penalty:':<40} {clf_en_LR.score(X, y):.2f}")
print(f"{'Score with L2 penalty:':<40} {clf_l2_LR.score(X, y):.2f}")
if i == 0:
axes_row[0].set_title("L1 penalty")
axes_row[1].set_title("Elastic-Net\nl1_ratio = %s" % l1_ratio)
axes_row[2].set_title("L2 penalty")
for ax, coefs in zip(axes_row, [coef_l1_LR, coef_en_LR, coef_l2_LR]):
ax.imshow(
np.abs(coefs.reshape(8, 8)),
interpolation="nearest",
cmap="binary",
vmax=1,
vmin=0,
)
ax.set_xticks(())
ax.set_yticks(())
axes_row[0].set_ylabel(f"C = {C}")
plt.show()
脚本总运行时间:(0分0.528秒)
相关示例