注意
转到结尾 下载完整的示例代码。或通过 JupyterLite 或 Binder 在您的浏览器中运行此示例
平衡模型复杂度和交叉验证得分#
此示例通过在最佳准确度得分的 1 个标准差范围内找到不错的准确度,同时最小化 PCA 分量的数量来平衡模型复杂度和交叉验证得分 [1]。
该图显示了交叉验证得分和 PCA 分量数量之间的权衡。当 n_components=10 且 accuracy=0.88 时,平衡的情况属于最佳准确度得分 1 个标准差范围内的范围。
[1] Hastie, T., Tibshirani, R., Friedman, J. (2001)。模型评估和选择。统计学习的要素(第 219-260 页)。纽约,纽约,美国:施普林格纽约公司。
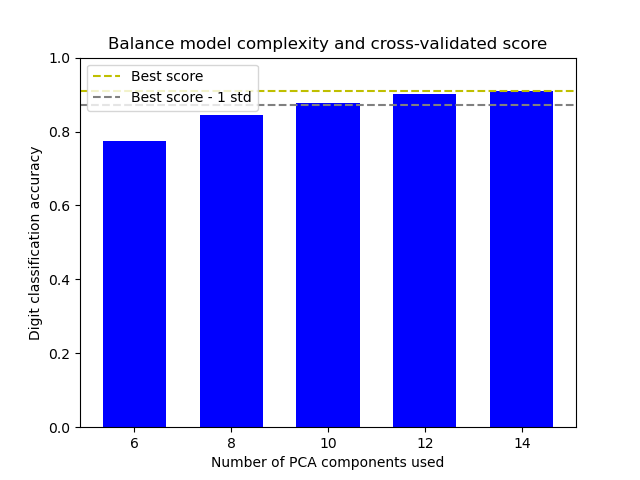
The best_index_ is 2
The n_components selected is 10
The corresponding accuracy score is 0.88
# Authors: The scikit-learn developers
# SPDX-License-Identifier: BSD-3-Clause
import matplotlib.pyplot as plt
import numpy as np
from sklearn.datasets import load_digits
from sklearn.decomposition import PCA
from sklearn.model_selection import GridSearchCV
from sklearn.pipeline import Pipeline
from sklearn.svm import LinearSVC
def lower_bound(cv_results):
"""
Calculate the lower bound within 1 standard deviation
of the best `mean_test_scores`.
Parameters
----------
cv_results : dict of numpy(masked) ndarrays
See attribute cv_results_ of `GridSearchCV`
Returns
-------
float
Lower bound within 1 standard deviation of the
best `mean_test_score`.
"""
best_score_idx = np.argmax(cv_results["mean_test_score"])
return (
cv_results["mean_test_score"][best_score_idx]
- cv_results["std_test_score"][best_score_idx]
)
def best_low_complexity(cv_results):
"""
Balance model complexity with cross-validated score.
Parameters
----------
cv_results : dict of numpy(masked) ndarrays
See attribute cv_results_ of `GridSearchCV`.
Return
------
int
Index of a model that has the fewest PCA components
while has its test score within 1 standard deviation of the best
`mean_test_score`.
"""
threshold = lower_bound(cv_results)
candidate_idx = np.flatnonzero(cv_results["mean_test_score"] >= threshold)
best_idx = candidate_idx[
cv_results["param_reduce_dim__n_components"][candidate_idx].argmin()
]
return best_idx
pipe = Pipeline(
[
("reduce_dim", PCA(random_state=42)),
("classify", LinearSVC(random_state=42, C=0.01)),
]
)
param_grid = {"reduce_dim__n_components": [6, 8, 10, 12, 14]}
grid = GridSearchCV(
pipe,
cv=10,
n_jobs=1,
param_grid=param_grid,
scoring="accuracy",
refit=best_low_complexity,
)
X, y = load_digits(return_X_y=True)
grid.fit(X, y)
n_components = grid.cv_results_["param_reduce_dim__n_components"]
test_scores = grid.cv_results_["mean_test_score"]
plt.figure()
plt.bar(n_components, test_scores, width=1.3, color="b")
lower = lower_bound(grid.cv_results_)
plt.axhline(np.max(test_scores), linestyle="--", color="y", label="Best score")
plt.axhline(lower, linestyle="--", color=".5", label="Best score - 1 std")
plt.title("Balance model complexity and cross-validated score")
plt.xlabel("Number of PCA components used")
plt.ylabel("Digit classification accuracy")
plt.xticks(n_components.tolist())
plt.ylim((0, 1.0))
plt.legend(loc="upper left")
best_index_ = grid.best_index_
print("The best_index_ is %d" % best_index_)
print("The n_components selected is %d" % n_components[best_index_])
print(
"The corresponding accuracy score is %.2f"
% grid.cv_results_["mean_test_score"][best_index_]
)
plt.show()
脚本的总运行时间:(0 分钟 1.265 秒)
相关示例