注意
转到末尾 下载完整的示例代码。或通过 JupyterLite 或 Binder 在您的浏览器中运行此示例
目标编码器的内部交叉拟合#
TargetEncoder
将分类特征的每个类别替换为该类别的目标变量的收缩均值。此方法在分类特征与目标之间存在强相关关系的情况下很有用。为了防止过拟合,TargetEncoder.fit_transform
使用内部交叉拟合方案来编码训练数据,供下游模型使用。此方案包括将数据分成 *k* 折,并使用其他 *k-1* 折学习的编码来编码每个折。在此示例中,我们演示了交叉拟合过程对于防止过拟合的重要性。
# Authors: The scikit-learn developers
# SPDX-License-Identifier: BSD-3-Clause
创建合成数据集#
在此示例中,我们构建一个具有三个分类特征的数据集
一个具有中等基数的信息特征(“信息性”)
一个具有中等基数的无信息特征(“随机”)
一个具有高基数的无信息特征(“近唯一”)
首先,我们生成信息特征
import numpy as np
from sklearn.preprocessing import KBinsDiscretizer
n_samples = 50_000
rng = np.random.RandomState(42)
y = rng.randn(n_samples)
noise = 0.5 * rng.randn(n_samples)
n_categories = 100
kbins = KBinsDiscretizer(
n_bins=n_categories,
encode="ordinal",
strategy="uniform",
random_state=rng,
subsample=None,
)
X_informative = kbins.fit_transform((y + noise).reshape(-1, 1))
# Remove the linear relationship between y and the bin index by permuting the
# values of X_informative:
permuted_categories = rng.permutation(n_categories)
X_informative = permuted_categories[X_informative.astype(np.int32)]
具有中等基数的无信息特征是通过置换信息特征并消除与目标的关系生成的
X_shuffled = rng.permutation(X_informative)
具有高基数的无信息特征的生成方式使其独立于目标变量。我们将展示,如果没有交叉拟合,目标编码将导致下游回归器的灾难性过拟合。这些高基数特征基本上是样本的唯一标识符,通常应从机器学习数据集中删除。在此示例中,我们生成它们以展示TargetEncoder
的默认交叉拟合行为如何自动减轻过拟合问题。
X_near_unique_categories = rng.choice(
int(0.9 * n_samples), size=n_samples, replace=True
).reshape(-1, 1)
最后,我们组装数据集并执行训练测试分割
import pandas as pd
from sklearn.model_selection import train_test_split
X = pd.DataFrame(
np.concatenate(
[X_informative, X_shuffled, X_near_unique_categories],
axis=1,
),
columns=["informative", "shuffled", "near_unique"],
)
X_train, X_test, y_train, y_test = train_test_split(X, y, random_state=0)
训练岭回归器#
在本节中,我们在具有和不具有编码的数据集上训练岭回归器,并探讨具有和不具有内部交叉拟合的目标编码器的影响。首先,我们看到在原始特征上训练的岭模型性能较低。这是因为我们置换了信息特征的顺序,这意味着X_informative
在原始状态下没有信息量
import sklearn
from sklearn.linear_model import Ridge
# Configure transformers to always output DataFrames
sklearn.set_config(transform_output="pandas")
ridge = Ridge(alpha=1e-6, solver="lsqr", fit_intercept=False)
raw_model = ridge.fit(X_train, y_train)
print("Raw Model score on training set: ", raw_model.score(X_train, y_train))
print("Raw Model score on test set: ", raw_model.score(X_test, y_test))
Raw Model score on training set: 0.0049896314219659565
Raw Model score on test set: 0.004577621581492997
接下来,我们创建一个带有目标编码器和岭模型的管道。该管道使用TargetEncoder.fit_transform
,它使用交叉拟合。我们看到模型很好地拟合了数据并泛化到测试集
from sklearn.pipeline import make_pipeline
from sklearn.preprocessing import TargetEncoder
model_with_cf = make_pipeline(TargetEncoder(random_state=0), ridge)
model_with_cf.fit(X_train, y_train)
print("Model with CF on train set: ", model_with_cf.score(X_train, y_train))
print("Model with CF on test set: ", model_with_cf.score(X_test, y_test))
Model with CF on train set: 0.8000184677460305
Model with CF on test set: 0.7927845601690917
线性模型的系数表明,大部分权重都在列索引为 0 的特征上,该特征是信息特征
import matplotlib.pyplot as plt
import pandas as pd
plt.rcParams["figure.constrained_layout.use"] = True
coefs_cf = pd.Series(
model_with_cf[-1].coef_, index=model_with_cf[-1].feature_names_in_
).sort_values()
ax = coefs_cf.plot(kind="barh")
_ = ax.set(
title="Target encoded with cross fitting",
xlabel="Ridge coefficient",
ylabel="Feature",
)
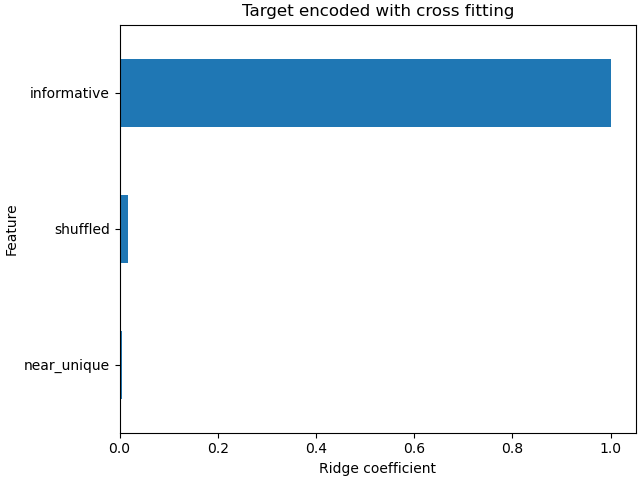
虽然TargetEncoder.fit_transform
使用内部交叉拟合方案来学习训练集的编码,但TargetEncoder.transform
本身不使用。它使用完整的训练集来学习编码并转换分类特征。因此,我们可以使用TargetEncoder.fit
然后使用TargetEncoder.transform
来禁用交叉拟合。然后,此编码将传递给岭模型。
target_encoder = TargetEncoder(random_state=0)
target_encoder.fit(X_train, y_train)
X_train_no_cf_encoding = target_encoder.transform(X_train)
X_test_no_cf_encoding = target_encoder.transform(X_test)
model_no_cf = ridge.fit(X_train_no_cf_encoding, y_train)
我们评估在编码时未使用交叉拟合的模型,并发现它过拟合了
print(
"Model without CF on training set: ",
model_no_cf.score(X_train_no_cf_encoding, y_train),
)
print(
"Model without CF on test set: ",
model_no_cf.score(
X_test_no_cf_encoding,
y_test,
),
)
Model without CF on training set: 0.858486250088675
Model without CF on test set: 0.6338211367102258
岭模型过拟合是因为与使用交叉拟合对特征进行编码的模型相比,它为无信息且基数极高的(“近唯一”)和中等基数(“随机”)特征分配了更大的权重。
coefs_no_cf = pd.Series(
model_no_cf.coef_, index=model_no_cf.feature_names_in_
).sort_values()
ax = coefs_no_cf.plot(kind="barh")
_ = ax.set(
title="Target encoded without cross fitting",
xlabel="Ridge coefficient",
ylabel="Feature",
)
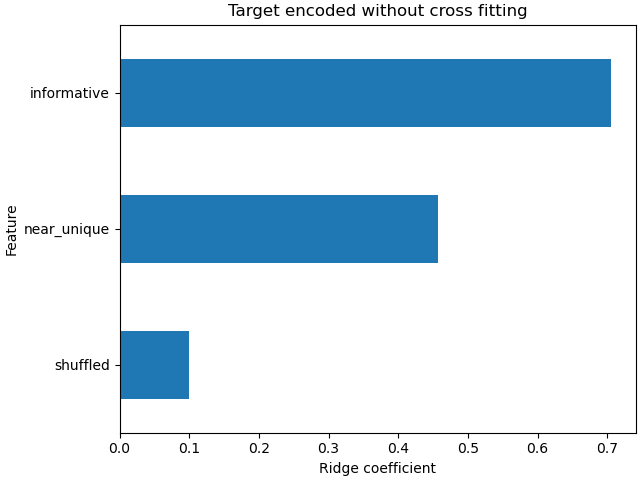
结论#
此示例演示了TargetEncoder
内部交叉拟合的重要性。在将训练数据传递给机器学习模型之前,务必使用TargetEncoder.fit_transform
对其进行编码。当TargetEncoder
是Pipeline
的一部分,并且管道已拟合时,管道将正确调用TargetEncoder.fit_transform
并在编码训练数据时使用交叉拟合。
脚本总运行时间:(0 分钟 0.315 秒)
相关示例