注意
转到末尾 下载完整的示例代码。或者通过JupyterLite或Binder在您的浏览器中运行此示例
K-Means和MiniBatchKMeans聚类算法的比较#
我们想比较MiniBatchKMeans和KMeans的性能:MiniBatchKMeans速度更快,但结果略有不同(参见Mini Batch K-Means)。
我们将首先使用KMeans,然后使用MiniBatchKMeans对一组数据进行聚类,并绘制结果。我们还将绘制两种算法之间标记不同的点。
# Authors: The scikit-learn developers
# SPDX-License-Identifier: BSD-3-Clause
生成数据#
我们首先生成要聚类的数据块。
import numpy as np
from sklearn.datasets import make_blobs
np.random.seed(0)
batch_size = 45
centers = [[1, 1], [-1, -1], [1, -1]]
n_clusters = len(centers)
X, labels_true = make_blobs(n_samples=3000, centers=centers, cluster_std=0.7)
使用KMeans计算聚类#
使用MiniBatchKMeans计算聚类#
from sklearn.cluster import MiniBatchKMeans
mbk = MiniBatchKMeans(
init="k-means++",
n_clusters=3,
batch_size=batch_size,
n_init=10,
max_no_improvement=10,
verbose=0,
)
t0 = time.time()
mbk.fit(X)
t_mini_batch = time.time() - t0
建立集群之间的同等性#
我们希望MiniBatchKMeans和KMeans算法具有相同聚类的相同颜色。让我们根据最接近的中心对聚类中心进行配对。
from sklearn.metrics.pairwise import pairwise_distances_argmin
k_means_cluster_centers = k_means.cluster_centers_
order = pairwise_distances_argmin(k_means.cluster_centers_, mbk.cluster_centers_)
mbk_means_cluster_centers = mbk.cluster_centers_[order]
k_means_labels = pairwise_distances_argmin(X, k_means_cluster_centers)
mbk_means_labels = pairwise_distances_argmin(X, mbk_means_cluster_centers)
绘制结果#
import matplotlib.pyplot as plt
fig = plt.figure(figsize=(8, 3))
fig.subplots_adjust(left=0.02, right=0.98, bottom=0.05, top=0.9)
colors = ["#4EACC5", "#FF9C34", "#4E9A06"]
# KMeans
ax = fig.add_subplot(1, 3, 1)
for k, col in zip(range(n_clusters), colors):
my_members = k_means_labels == k
cluster_center = k_means_cluster_centers[k]
ax.plot(X[my_members, 0], X[my_members, 1], "w", markerfacecolor=col, marker=".")
ax.plot(
cluster_center[0],
cluster_center[1],
"o",
markerfacecolor=col,
markeredgecolor="k",
markersize=6,
)
ax.set_title("KMeans")
ax.set_xticks(())
ax.set_yticks(())
plt.text(-3.5, 1.8, "train time: %.2fs\ninertia: %f" % (t_batch, k_means.inertia_))
# MiniBatchKMeans
ax = fig.add_subplot(1, 3, 2)
for k, col in zip(range(n_clusters), colors):
my_members = mbk_means_labels == k
cluster_center = mbk_means_cluster_centers[k]
ax.plot(X[my_members, 0], X[my_members, 1], "w", markerfacecolor=col, marker=".")
ax.plot(
cluster_center[0],
cluster_center[1],
"o",
markerfacecolor=col,
markeredgecolor="k",
markersize=6,
)
ax.set_title("MiniBatchKMeans")
ax.set_xticks(())
ax.set_yticks(())
plt.text(-3.5, 1.8, "train time: %.2fs\ninertia: %f" % (t_mini_batch, mbk.inertia_))
# Initialize the different array to all False
different = mbk_means_labels == 4
ax = fig.add_subplot(1, 3, 3)
for k in range(n_clusters):
different += (k_means_labels == k) != (mbk_means_labels == k)
identical = np.logical_not(different)
ax.plot(X[identical, 0], X[identical, 1], "w", markerfacecolor="#bbbbbb", marker=".")
ax.plot(X[different, 0], X[different, 1], "w", markerfacecolor="m", marker=".")
ax.set_title("Difference")
ax.set_xticks(())
ax.set_yticks(())
plt.show()
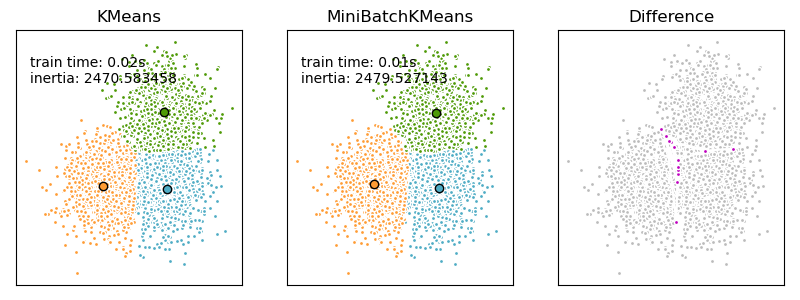
脚本总运行时间:(0分0.210秒)
相关示例