注意
转到结尾 下载完整的示例代码。或者通过 JupyterLite 或 Binder 在浏览器中运行此示例
使用多项逻辑回归 + L1 的 MNIST 分类#
在这里,我们使用 L1 惩罚在 MNIST 数字分类任务的子集上拟合多项逻辑回归。为此,我们使用 SAGA 算法:这是一种在样本数量远大于特征数量时速度很快的求解器,并且能够很好地优化非平滑目标函数,而这正是 l1 惩罚的情况。测试准确率达到 > 0.8,而权重向量保持稀疏,因此更容易解释。
请注意,这种 l1 惩罚线性模型的准确率远低于 l2 惩罚线性模型或非线性多层感知器模型在此数据集上所能达到的准确率。
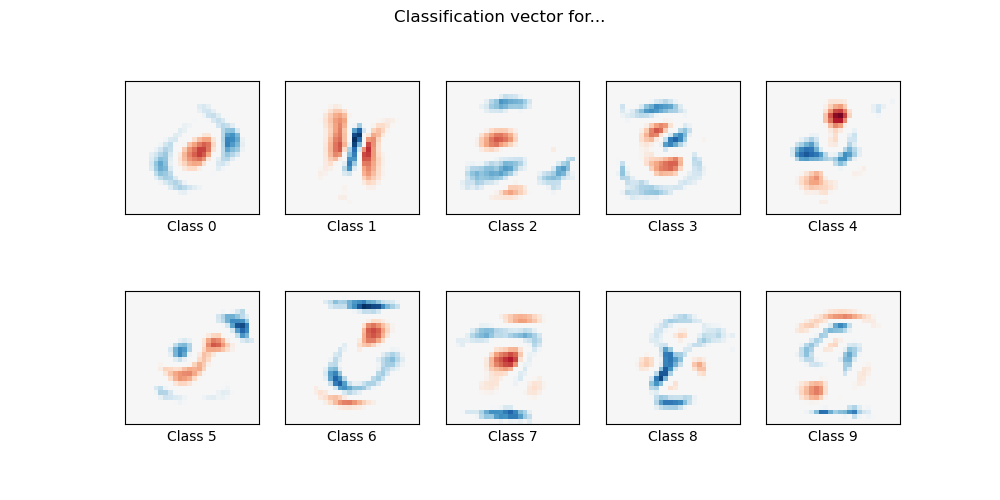
Sparsity with L1 penalty: 74.57%
Test score with L1 penalty: 0.8253
Example run in 8.308 s
# Authors: The scikit-learn developers
# SPDX-License-Identifier: BSD-3-Clause
import time
import matplotlib.pyplot as plt
import numpy as np
from sklearn.datasets import fetch_openml
from sklearn.linear_model import LogisticRegression
from sklearn.model_selection import train_test_split
from sklearn.preprocessing import StandardScaler
from sklearn.utils import check_random_state
# Turn down for faster convergence
t0 = time.time()
train_samples = 5000
# Load data from https://www.openml.org/d/554
X, y = fetch_openml("mnist_784", version=1, return_X_y=True, as_frame=False)
random_state = check_random_state(0)
permutation = random_state.permutation(X.shape[0])
X = X[permutation]
y = y[permutation]
X = X.reshape((X.shape[0], -1))
X_train, X_test, y_train, y_test = train_test_split(
X, y, train_size=train_samples, test_size=10000
)
scaler = StandardScaler()
X_train = scaler.fit_transform(X_train)
X_test = scaler.transform(X_test)
# Turn up tolerance for faster convergence
clf = LogisticRegression(C=50.0 / train_samples, penalty="l1", solver="saga", tol=0.1)
clf.fit(X_train, y_train)
sparsity = np.mean(clf.coef_ == 0) * 100
score = clf.score(X_test, y_test)
# print('Best C % .4f' % clf.C_)
print("Sparsity with L1 penalty: %.2f%%" % sparsity)
print("Test score with L1 penalty: %.4f" % score)
coef = clf.coef_.copy()
plt.figure(figsize=(10, 5))
scale = np.abs(coef).max()
for i in range(10):
l1_plot = plt.subplot(2, 5, i + 1)
l1_plot.imshow(
coef[i].reshape(28, 28),
interpolation="nearest",
cmap=plt.cm.RdBu,
vmin=-scale,
vmax=scale,
)
l1_plot.set_xticks(())
l1_plot.set_yticks(())
l1_plot.set_xlabel("Class %i" % i)
plt.suptitle("Classification vector for...")
run_time = time.time() - t0
print("Example run in %.3f s" % run_time)
plt.show()
脚本总运行时间:(0 分钟 8.377 秒)
相关示例