注意
前往末尾下载完整的示例代码,或通过JupyterLite或Binder在浏览器中运行此示例。
scikit-learn 1.1版本亮点#
我们很高兴地宣布scikit-learn 1.1版本的发布!添加了许多错误修复和改进,以及一些新的关键特性。我们在下面详细介绍了此版本的一些主要功能。**有关所有更改的详尽列表**,请参阅发行说明。
要安装最新版本(使用pip)
pip install --upgrade scikit-learn
或使用conda
conda install -c conda-forge scikit-learn
分位数损失在 HistGradientBoostingRegressor
#
HistGradientBoostingRegressor
可以使用 loss="quantile"
和新参数 quantile
来建模分位数。
from sklearn.ensemble import HistGradientBoostingRegressor
import numpy as np
import matplotlib.pyplot as plt
# Simple regression function for X * cos(X)
rng = np.random.RandomState(42)
X_1d = np.linspace(0, 10, num=2000)
X = X_1d.reshape(-1, 1)
y = X_1d * np.cos(X_1d) + rng.normal(scale=X_1d / 3)
quantiles = [0.95, 0.5, 0.05]
parameters = dict(loss="quantile", max_bins=32, max_iter=50)
hist_quantiles = {
f"quantile={quantile:.2f}": HistGradientBoostingRegressor(
**parameters, quantile=quantile
).fit(X, y)
for quantile in quantiles
}
fig, ax = plt.subplots()
ax.plot(X_1d, y, "o", alpha=0.5, markersize=1)
for quantile, hist in hist_quantiles.items():
ax.plot(X_1d, hist.predict(X), label=quantile)
_ = ax.legend(loc="lower left")
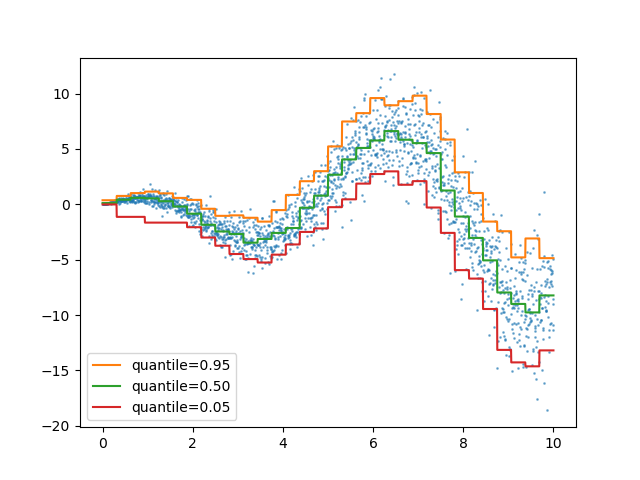
有关用例示例,请参见 直方图梯度提升树中的特征
get_feature_names_out
可用于所有转换器#
get_feature_names_out 现在可用于所有转换器。这使得 Pipeline
能够为更复杂的管道构建输出特征名称。
from sklearn.compose import ColumnTransformer
from sklearn.preprocessing import OneHotEncoder, StandardScaler
from sklearn.pipeline import make_pipeline
from sklearn.impute import SimpleImputer
from sklearn.feature_selection import SelectKBest
from sklearn.datasets import fetch_openml
from sklearn.linear_model import LogisticRegression
X, y = fetch_openml(
"titanic", version=1, as_frame=True, return_X_y=True, parser="pandas"
)
numeric_features = ["age", "fare"]
numeric_transformer = make_pipeline(SimpleImputer(strategy="median"), StandardScaler())
categorical_features = ["embarked", "pclass"]
preprocessor = ColumnTransformer(
[
("num", numeric_transformer, numeric_features),
(
"cat",
OneHotEncoder(handle_unknown="ignore", sparse_output=False),
categorical_features,
),
],
verbose_feature_names_out=False,
)
log_reg = make_pipeline(preprocessor, SelectKBest(k=7), LogisticRegression())
log_reg.fit(X, y)
在这里,我们将管道切片以包含除最后一个步骤之外的所有步骤。此管道切片的输出特征名称是放入逻辑回归中的特征。这些名称直接对应于逻辑回归中的系数。
import pandas as pd
log_reg_input_features = log_reg[:-1].get_feature_names_out()
pd.Series(log_reg[-1].coef_.ravel(), index=log_reg_input_features).plot.bar()
plt.tight_layout()
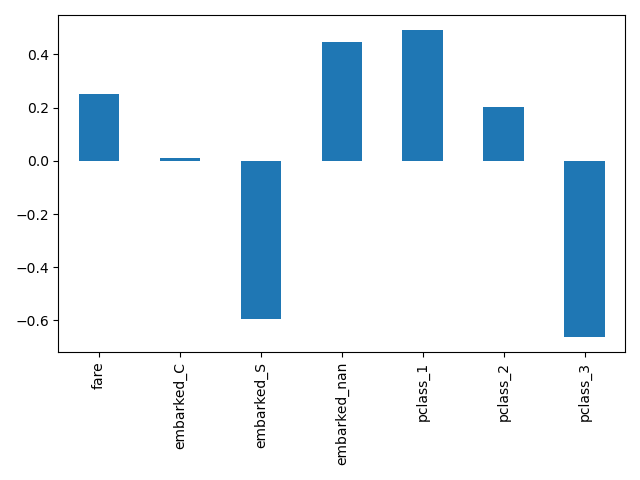
在 OneHotEncoder
中分组不常见类别#
OneHotEncoder
支持将不常见类别聚合到每个特征的单个输出中。启用收集不常见类别的参数是 min_frequency
和 max_categories
。有关更多详细信息,请参见 用户指南。
from sklearn.preprocessing import OneHotEncoder
import numpy as np
X = np.array(
[["dog"] * 5 + ["cat"] * 20 + ["rabbit"] * 10 + ["snake"] * 3], dtype=object
).T
enc = OneHotEncoder(min_frequency=6, sparse_output=False).fit(X)
enc.infrequent_categories_
[array(['dog', 'snake'], dtype=object)]
由于狗和蛇是不常见的类别,因此在转换时将它们组合在一起。
encoded = enc.transform(np.array([["dog"], ["snake"], ["cat"], ["rabbit"]]))
pd.DataFrame(encoded, columns=enc.get_feature_names_out())
性能改进#
针对密集型 float64 数据集的成对距离减少已重构,以更好地利用非阻塞线程并行性。例如,neighbors.NearestNeighbors.kneighbors
和 neighbors.NearestNeighbors.radius_neighbors
的速度分别比以前快达 ×20 和 ×5。总而言之,以下函数和估计器现在受益于改进的性能:
要了解有关这项工作的技术细节的更多信息,您可以阅读 这篇博文系列。
此外,使用 Cython 重构了损失函数的计算,从而提高了以下估计器的性能:
MiniBatchNMF
:NMF 的在线版本#
新类 MiniBatchNMF
实现了一个更快但精度较低的非负矩阵分解版本(NMF
)。MiniBatchNMF
将数据分成小批量,并通过循环遍历小批量以在线方式优化 NMF 模型,使其更适合大型数据集。特别是,它实现了 partial_fit
,当数据并非一开始就 readily available 或数据无法放入内存中时,可用于在线学习。
import numpy as np
from sklearn.decomposition import MiniBatchNMF
rng = np.random.RandomState(0)
n_samples, n_features, n_components = 10, 10, 5
true_W = rng.uniform(size=(n_samples, n_components))
true_H = rng.uniform(size=(n_components, n_features))
X = true_W @ true_H
nmf = MiniBatchNMF(n_components=n_components, random_state=0)
for _ in range(10):
nmf.partial_fit(X)
W = nmf.transform(X)
H = nmf.components_
X_reconstructed = W @ H
print(
f"relative reconstruction error: ",
f"{np.sum((X - X_reconstructed) ** 2) / np.sum(X**2):.5f}",
)
relative reconstruction error: 0.00364
BisectingKMeans
:划分和聚类#
新类 BisectingKMeans
是 KMeans
的一个变体,使用分裂式层次聚类。它不是一次创建所有质心,而是根据之前的聚类逐步选择质心:一个聚类被反复分成两个新聚类,直到达到目标聚类数量,从而为聚类提供层次结构。
from sklearn.datasets import make_blobs
from sklearn.cluster import KMeans, BisectingKMeans
import matplotlib.pyplot as plt
X, _ = make_blobs(n_samples=1000, centers=2, random_state=0)
km = KMeans(n_clusters=5, random_state=0, n_init="auto").fit(X)
bisect_km = BisectingKMeans(n_clusters=5, random_state=0).fit(X)
fig, ax = plt.subplots(1, 2, figsize=(10, 5))
ax[0].scatter(X[:, 0], X[:, 1], s=10, c=km.labels_)
ax[0].scatter(km.cluster_centers_[:, 0], km.cluster_centers_[:, 1], s=20, c="r")
ax[0].set_title("KMeans")
ax[1].scatter(X[:, 0], X[:, 1], s=10, c=bisect_km.labels_)
ax[1].scatter(
bisect_km.cluster_centers_[:, 0], bisect_km.cluster_centers_[:, 1], s=20, c="r"
)
_ = ax[1].set_title("BisectingKMeans")
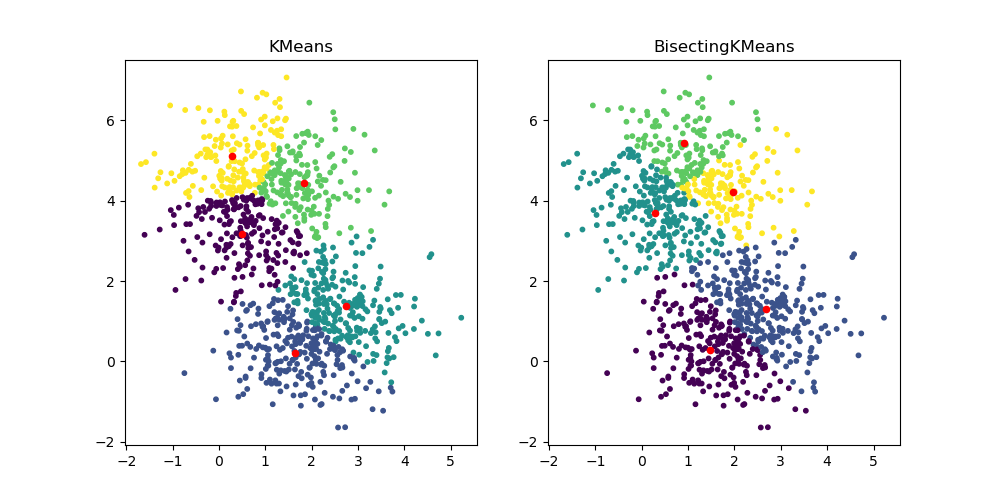
脚本总运行时间:(0 分钟 0.967 秒)
相关示例