注意
点击此处 下载完整示例代码。或通过JupyterLite或Binder在浏览器中运行此示例。
特征缩放的重要性#
通过标准化进行的特征缩放,也称为Z分数标准化,是许多机器学习算法的重要预处理步骤。它涉及重新缩放每个特征,使其标准差为1,均值为0。
即使基于树的模型(几乎)不受缩放的影响,许多其他算法也需要对特征进行归一化,原因各不相同:为了简化收敛(例如非惩罚逻辑回归),或者为了创建与未缩放数据拟合相比完全不同的模型拟合(例如KNeighbors模型)。本例的第一部分演示了后者。
在本例的第二部分中,我们将展示主成分分析 (PCA) 如何受特征归一化的影响。为了说明这一点,我们将使用PCA
对未缩放数据找到的主成分与使用StandardScaler
首先缩放数据获得的主成分进行比较。
在本例的最后一部分中,我们将展示归一化对在PCA降维数据上训练的模型精度的影响。
# Authors: The scikit-learn developers
# SPDX-License-Identifier: BSD-3-Clause
加载和准备数据#
使用的数据集是UCI提供的葡萄酒识别数据集。此数据集具有连续特征,由于测量属性的不同(例如酒精含量和苹果酸),这些特征的规模不均一。
from sklearn.datasets import load_wine
from sklearn.model_selection import train_test_split
from sklearn.preprocessing import StandardScaler
X, y = load_wine(return_X_y=True, as_frame=True)
scaler = StandardScaler().set_output(transform="pandas")
X_train, X_test, y_train, y_test = train_test_split(
X, y, test_size=0.30, random_state=42
)
scaled_X_train = scaler.fit_transform(X_train)
重新缩放对k-近邻模型的影响#
为了可视化KNeighborsClassifier
的决策边界,在本节中,我们选择具有不同数量级值的两个特征的子集。
请记住,使用特征子集训练模型可能会遗漏具有高预测影响的特征,导致决策边界与在完整特征集上训练的模型相比要差得多。
import matplotlib.pyplot as plt
from sklearn.inspection import DecisionBoundaryDisplay
from sklearn.neighbors import KNeighborsClassifier
X_plot = X[["proline", "hue"]]
X_plot_scaled = scaler.fit_transform(X_plot)
clf = KNeighborsClassifier(n_neighbors=20)
def fit_and_plot_model(X_plot, y, clf, ax):
clf.fit(X_plot, y)
disp = DecisionBoundaryDisplay.from_estimator(
clf,
X_plot,
response_method="predict",
alpha=0.5,
ax=ax,
)
disp.ax_.scatter(X_plot["proline"], X_plot["hue"], c=y, s=20, edgecolor="k")
disp.ax_.set_xlim((X_plot["proline"].min(), X_plot["proline"].max()))
disp.ax_.set_ylim((X_plot["hue"].min(), X_plot["hue"].max()))
return disp.ax_
fig, (ax1, ax2) = plt.subplots(ncols=2, figsize=(12, 6))
fit_and_plot_model(X_plot, y, clf, ax1)
ax1.set_title("KNN without scaling")
fit_and_plot_model(X_plot_scaled, y, clf, ax2)
ax2.set_xlabel("scaled proline")
ax2.set_ylabel("scaled hue")
_ = ax2.set_title("KNN with scaling")
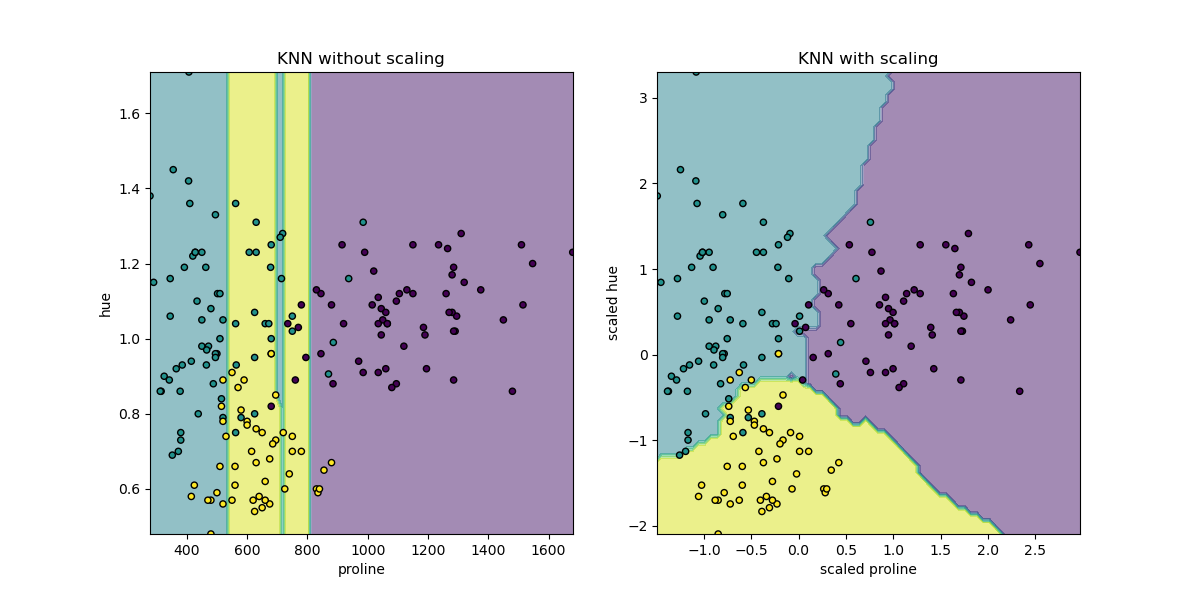
此处决策边界表明,拟合缩放或未缩放的数据会导致完全不同的模型。原因是变量“脯氨酸”的值在0到1000之间变化;而变量“色调”在1到10之间变化。因此,样本间的距离主要受“脯氨酸”值差异的影响,而“色调”的值则会被相对忽略。如果使用StandardScaler
来归一化此数据库,则缩放后的值都大致在-3到3之间,并且邻居结构将或多或少地受到这两个变量的同等影响。
重新缩放对PCA降维的影响#
使用PCA
进行降维包括寻找最大化方差的特征。如果一个特征的变化幅度大于其他特征,仅仅是因为它们各自的尺度不同,PCA
将确定此类特征主导主成分的方向。
我们可以使用所有原始特征检查第一个主成分。
import pandas as pd
from sklearn.decomposition import PCA
pca = PCA(n_components=2).fit(X_train)
scaled_pca = PCA(n_components=2).fit(scaled_X_train)
X_train_transformed = pca.transform(X_train)
X_train_std_transformed = scaled_pca.transform(scaled_X_train)
first_pca_component = pd.DataFrame(
pca.components_[0], index=X.columns, columns=["without scaling"]
)
first_pca_component["with scaling"] = scaled_pca.components_[0]
first_pca_component.plot.bar(
title="Weights of the first principal component", figsize=(6, 8)
)
_ = plt.tight_layout()
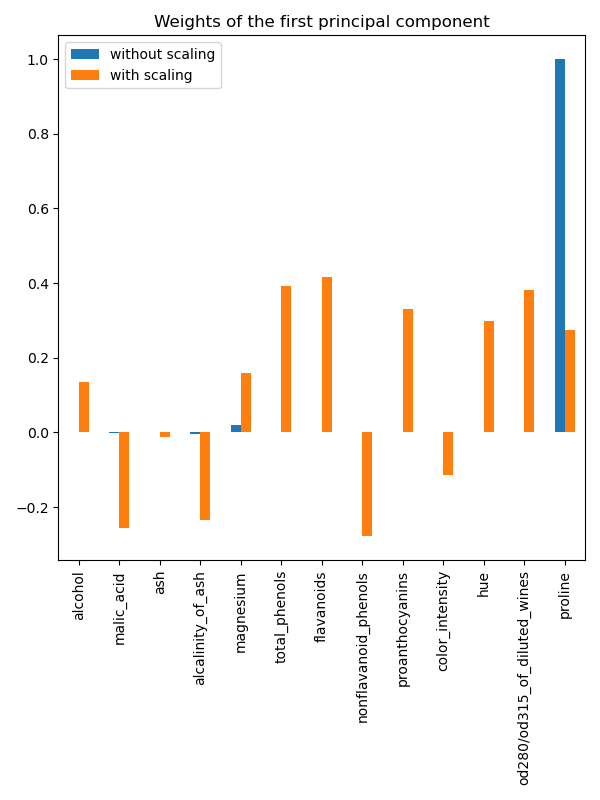
事实上,我们发现如果没有缩放,“脯氨酸”特征主导第一个主成分的方向,其数量级比其他特征高出约两个数量级。这与观察数据缩放版本中的第一个主成分时形成对比,其中所有特征的数量级大致相同。
我们可以将这两种情况下主成分的分布可视化。
fig, (ax1, ax2) = plt.subplots(nrows=1, ncols=2, figsize=(10, 5))
target_classes = range(0, 3)
colors = ("blue", "red", "green")
markers = ("^", "s", "o")
for target_class, color, marker in zip(target_classes, colors, markers):
ax1.scatter(
x=X_train_transformed[y_train == target_class, 0],
y=X_train_transformed[y_train == target_class, 1],
color=color,
label=f"class {target_class}",
alpha=0.5,
marker=marker,
)
ax2.scatter(
x=X_train_std_transformed[y_train == target_class, 0],
y=X_train_std_transformed[y_train == target_class, 1],
color=color,
label=f"class {target_class}",
alpha=0.5,
marker=marker,
)
ax1.set_title("Unscaled training dataset after PCA")
ax2.set_title("Standardized training dataset after PCA")
for ax in (ax1, ax2):
ax.set_xlabel("1st principal component")
ax.set_ylabel("2nd principal component")
ax.legend(loc="upper right")
ax.grid()
_ = plt.tight_layout()
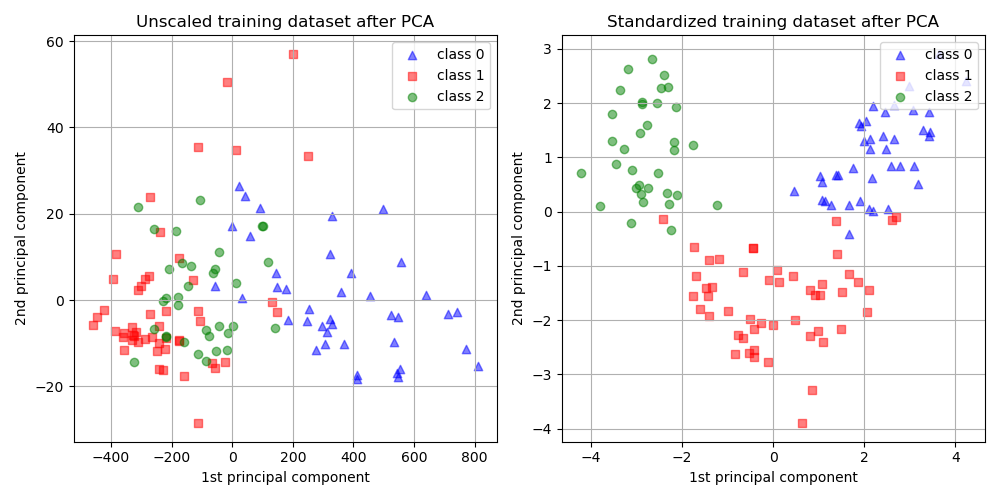
从上图可以看出,在降维之前缩放特征会导致分量具有相同数量级。在这种情况下,它还可以提高类的可分离性。事实上,在下一节中,我们将确认更好的可分离性对整体模型的性能有良好的影响。
重新缩放对模型性能的影响#
首先,我们将展示LogisticRegressionCV
的最佳正则化如何取决于数据的缩放或未缩放。
import numpy as np
from sklearn.linear_model import LogisticRegressionCV
from sklearn.pipeline import make_pipeline
Cs = np.logspace(-5, 5, 20)
unscaled_clf = make_pipeline(pca, LogisticRegressionCV(Cs=Cs))
unscaled_clf.fit(X_train, y_train)
scaled_clf = make_pipeline(scaler, pca, LogisticRegressionCV(Cs=Cs))
scaled_clf.fit(X_train, y_train)
print(f"Optimal C for the unscaled PCA: {unscaled_clf[-1].C_[0]:.4f}\n")
print(f"Optimal C for the standardized data with PCA: {scaled_clf[-1].C_[0]:.2f}")
Optimal C for the unscaled PCA: 0.0004
Optimal C for the standardized data with PCA: 20.69
对于在应用 PCA 之前未缩放的数据,正则化的需求更高(C
的值较低)。我们现在评估缩放对最佳模型的准确性和平均对数损失的影响。
from sklearn.metrics import accuracy_score, log_loss
y_pred = unscaled_clf.predict(X_test)
y_pred_scaled = scaled_clf.predict(X_test)
y_proba = unscaled_clf.predict_proba(X_test)
y_proba_scaled = scaled_clf.predict_proba(X_test)
print("Test accuracy for the unscaled PCA")
print(f"{accuracy_score(y_test, y_pred):.2%}\n")
print("Test accuracy for the standardized data with PCA")
print(f"{accuracy_score(y_test, y_pred_scaled):.2%}\n")
print("Log-loss for the unscaled PCA")
print(f"{log_loss(y_test, y_proba):.3}\n")
print("Log-loss for the standardized data with PCA")
print(f"{log_loss(y_test, y_proba_scaled):.3}")
Test accuracy for the unscaled PCA
35.19%
Test accuracy for the standardized data with PCA
96.30%
Log-loss for the unscaled PCA
0.957
Log-loss for the standardized data with PCA
0.0825
当在PCA
之前缩放数据时,观察到预测精度存在明显差异,因为它大大优于未缩放的版本。这与上一节图中获得的直觉相对应,其中分量在使用PCA
之前缩放时变得线性可分离。
请注意,在这种情况下,具有缩放特征的模型的性能优于具有未缩放特征的模型,因为所有变量都应具有预测性,我们宁愿避免某些变量被相对忽略。
如果较小尺度的变量没有预测性,则在缩放特征后可能会出现性能下降:噪声特征在缩放后会对预测贡献更多,因此缩放会增加过拟合。
最后但并非最不重要的一点是,我们观察到通过缩放步骤可以实现更低的对数损失。
脚本总运行时间:(0 分钟 1.966 秒)
相关示例