注意
转到结尾 下载完整的示例代码。或者通过JupyterLite或Binder在浏览器中运行此示例
时间序列预测的滞后特征#
此示例演示了如何使用Polars生成的滞后特征以及HistGradientBoostingRegressor
对共享单车需求数据集进行时间序列预测。
请参阅关于时间相关特征工程的示例,了解有关此数据集的一些数据探索以及周期性特征工程的演示。
# Authors: The scikit-learn developers
# SPDX-License-Identifier: BSD-3-Clause
分析共享单车需求数据集#
我们首先从OpenML存储库加载数据作为原始parquet文件,以说明如何使用任意parquet文件,而不是将此步骤隐藏在诸如sklearn.datasets.fetch_openml
之类的便捷工具中。
parquet文件的URL可以在openml.org上ID为44063的共享单车需求数据集的JSON描述中找到(https://openml.org/search?type=data&status=active&id=44063)。
还提供了文件的sha256
哈希值,以确保下载文件的完整性。
import numpy as np
import polars as pl
from sklearn.datasets import fetch_file
pl.Config.set_fmt_str_lengths(20)
bike_sharing_data_file = fetch_file(
"https://openml1.win.tue.nl/datasets/0004/44063/dataset_44063.pq",
sha256="d120af76829af0d256338dc6dd4be5df4fd1f35bf3a283cab66a51c1c6abd06a",
)
bike_sharing_data_file
PosixPath('/home/circleci/scikit_learn_data/openml1.win.tue.nl/datasets_0004_44063/dataset_44063.pq')
我们使用Polars加载parquet文件进行特征工程。Polars会自动缓存多个表达式中重复使用的常见子表达式(如下面的pl.col("count").shift(1)
)。有关更多信息,请参见https://docs.pola.rs/user-guide/lazy/optimizations/。
df = pl.read_parquet(bike_sharing_data_file)
接下来,我们查看数据集的统计摘要,以便更好地理解我们正在处理的数据。
import polars.selectors as cs
summary = df.select(cs.numeric()).describe()
summary
让我们看看数据集中存在的季节"秋季"
、"春季"
、"夏季"
和"冬季"
的数量,以确认它们是平衡的。
import matplotlib.pyplot as plt
df["season"].value_counts()
生成Polars生成的滞后特征#
让我们考虑一下根据过去的需求预测下一小时的需求的问题。由于需求是一个连续变量,因此直观地可以使用任何回归模型。但是,我们没有通常的(X_train, y_train)
数据集。相反,我们只是按时间顺序组织的y_train
需求数据。
lagged_df = df.select(
"count",
*[pl.col("count").shift(i).alias(f"lagged_count_{i}h") for i in [1, 2, 3]],
lagged_count_1d=pl.col("count").shift(24),
lagged_count_1d_1h=pl.col("count").shift(24 + 1),
lagged_count_7d=pl.col("count").shift(7 * 24),
lagged_count_7d_1h=pl.col("count").shift(7 * 24 + 1),
lagged_mean_24h=pl.col("count").shift(1).rolling_mean(24),
lagged_max_24h=pl.col("count").shift(1).rolling_max(24),
lagged_min_24h=pl.col("count").shift(1).rolling_min(24),
lagged_mean_7d=pl.col("count").shift(1).rolling_mean(7 * 24),
lagged_max_7d=pl.col("count").shift(1).rolling_max(7 * 24),
lagged_min_7d=pl.col("count").shift(1).rolling_min(7 * 24),
)
lagged_df.tail(10)
但是要注意,第一行有未定义的值,因为它们的过去是未知的。这取决于我们使用了多少滞后。
lagged_df.head(10)
现在,我们可以将滞后特征分离到矩阵X
中,并将目标变量(要预测的数量)分离到具有相同第一维度的数组y
中。
lagged_df = lagged_df.drop_nulls()
X = lagged_df.drop("count")
y = lagged_df["count"]
print("X shape: {}\ny shape: {}".format(X.shape, y.shape))
X shape: (17210, 13)
y shape: (17210,)
下一小时单车需求回归的简单评估#
让我们随机拆分我们的表格化数据集来训练梯度提升回归树 (GBRT) 模型,并使用平均绝对百分比误差 (MAPE) 对其进行评估。如果我们的模型旨在进行预测(即,根据过去的数据预测未来的数据),我们不应使用晚于测试数据的训练数据。在时间序列机器学习中,“i.i.d”(独立同分布)假设并不成立,因为数据点不是独立的,并且具有时间关系。
from sklearn.ensemble import HistGradientBoostingRegressor
from sklearn.model_selection import train_test_split
X_train, X_test, y_train, y_test = train_test_split(
X, y, test_size=0.2, random_state=42
)
model = HistGradientBoostingRegressor().fit(X_train, y_train)
查看模型的性能。
from sklearn.metrics import mean_absolute_percentage_error
y_pred = model.predict(X_test)
mean_absolute_percentage_error(y_test, y_pred)
0.3889873516666431
正确的下一小时预测评估#
让我们使用适当的评估拆分策略,该策略考虑了数据集的时间结构,以评估我们的模型预测未来数据点(避免通过读取训练集中滞后特征的值作弊)的能力。
from sklearn.model_selection import TimeSeriesSplit
ts_cv = TimeSeriesSplit(
n_splits=3, # to keep the notebook fast enough on common laptops
gap=48, # 2 days data gap between train and test
max_train_size=10000, # keep train sets of comparable sizes
test_size=3000, # for 2 or 3 digits of precision in scores
)
all_splits = list(ts_cv.split(X, y))
训练模型并根据MAPE评估其性能。
train_idx, test_idx = all_splits[0]
X_train, X_test = X[train_idx, :], X[test_idx, :]
y_train, y_test = y[train_idx], y[test_idx]
model = HistGradientBoostingRegressor().fit(X_train, y_train)
y_pred = model.predict(X_test)
mean_absolute_percentage_error(y_test, y_pred)
0.44300751539296973
通过随机训练测试拆分测量的泛化误差过于乐观。通过基于时间拆分进行的泛化更可能代表回归模型的真实性能。让我们用正确的交叉验证来评估这种误差评估的可变性。
from sklearn.model_selection import cross_val_score
cv_mape_scores = -cross_val_score(
model, X, y, cv=ts_cv, scoring="neg_mean_absolute_percentage_error"
)
cv_mape_scores
array([0.44300752, 0.27772182, 0.3697178 ])
拆分之间的可变性相当大!在现实环境中,建议使用更多拆分来更好地评估可变性。从现在开始,让我们报告平均CV分数及其标准差。
print(f"CV MAPE: {cv_mape_scores.mean():.3f} ± {cv_mape_scores.std():.3f}")
CV MAPE: 0.363 ± 0.068
我们可以计算评估指标和损失函数的几种组合,这些组合将在下面稍作报告。
from collections import defaultdict
from sklearn.metrics import (
make_scorer,
mean_absolute_error,
mean_pinball_loss,
root_mean_squared_error,
)
from sklearn.model_selection import cross_validate
def consolidate_scores(cv_results, scores, metric):
if metric == "MAPE":
scores[metric].append(f"{value.mean():.2f} ± {value.std():.2f}")
else:
scores[metric].append(f"{value.mean():.1f} ± {value.std():.1f}")
return scores
scoring = {
"MAPE": make_scorer(mean_absolute_percentage_error),
"RMSE": make_scorer(root_mean_squared_error),
"MAE": make_scorer(mean_absolute_error),
"pinball_loss_05": make_scorer(mean_pinball_loss, alpha=0.05),
"pinball_loss_50": make_scorer(mean_pinball_loss, alpha=0.50),
"pinball_loss_95": make_scorer(mean_pinball_loss, alpha=0.95),
}
loss_functions = ["squared_error", "poisson", "absolute_error"]
scores = defaultdict(list)
for loss_func in loss_functions:
model = HistGradientBoostingRegressor(loss=loss_func)
cv_results = cross_validate(
model,
X,
y,
cv=ts_cv,
scoring=scoring,
n_jobs=2,
)
time = cv_results["fit_time"]
scores["loss"].append(loss_func)
scores["fit_time"].append(f"{time.mean():.2f} ± {time.std():.2f} s")
for key, value in cv_results.items():
if key.startswith("test_"):
metric = key.split("test_")[1]
scores = consolidate_scores(cv_results, scores, metric)
通过分位数回归建模预测不确定性#
与其像最小二乘法和泊松损失那样对\(Y|X\)分布的期望值进行建模,不如尝试估计条件分布的分位数。
对于给定的数据点\(x_i\),\(Y|X=x_i\) 预计是一个随机变量,因为我们预计租赁数量无法从特征中完全准确地预测。它可能会受到现有滞后特征未充分捕获的其他变量的影响。例如,下一小时是否下雨无法完全从过去几小时的自行车租赁数据中预测。这就是我们所说的偶然不确定性。
分位数回归使得能够对该分布进行更精细的描述,而无需对其形状做出强假设。
quantile_list = [0.05, 0.5, 0.95]
for quantile in quantile_list:
model = HistGradientBoostingRegressor(loss="quantile", quantile=quantile)
cv_results = cross_validate(
model,
X,
y,
cv=ts_cv,
scoring=scoring,
n_jobs=2,
)
time = cv_results["fit_time"]
scores["fit_time"].append(f"{time.mean():.2f} ± {time.std():.2f} s")
scores["loss"].append(f"quantile {int(quantile*100)}")
for key, value in cv_results.items():
if key.startswith("test_"):
metric = key.split("test_")[1]
scores = consolidate_scores(cv_results, scores, metric)
scores_df = pl.DataFrame(scores)
scores_df
让我们看看最小化每个指标的损失。
def min_arg(col):
col_split = pl.col(col).str.split(" ")
return pl.arg_sort_by(
col_split.list.get(0).cast(pl.Float64),
col_split.list.get(2).cast(pl.Float64),
).first()
scores_df.select(
pl.col("loss").get(min_arg(col_name)).alias(col_name)
for col_name in scores_df.columns
if col_name != "loss"
)
即使由于数据集中的方差导致分数分布重叠,但当loss="squared_error"
时,平均RMSE较低,而当loss="absolute_error"
时,平均MAPE较低,这符合预期。对于分位数 5 和 95 的平均分位数损失也是如此。对应于 50 分位数损失的分数与通过最小化其他损失函数获得的分数重叠,MAE 也是如此。
定性地观察预测结果#
现在,我们可以根据第 5 个百分位数、中位数和第 95 个百分位数来可视化模型的性能。
all_splits = list(ts_cv.split(X, y))
train_idx, test_idx = all_splits[0]
X_train, X_test = X[train_idx, :], X[test_idx, :]
y_train, y_test = y[train_idx], y[test_idx]
max_iter = 50
gbrt_mean_poisson = HistGradientBoostingRegressor(loss="poisson", max_iter=max_iter)
gbrt_mean_poisson.fit(X_train, y_train)
mean_predictions = gbrt_mean_poisson.predict(X_test)
gbrt_median = HistGradientBoostingRegressor(
loss="quantile", quantile=0.5, max_iter=max_iter
)
gbrt_median.fit(X_train, y_train)
median_predictions = gbrt_median.predict(X_test)
gbrt_percentile_5 = HistGradientBoostingRegressor(
loss="quantile", quantile=0.05, max_iter=max_iter
)
gbrt_percentile_5.fit(X_train, y_train)
percentile_5_predictions = gbrt_percentile_5.predict(X_test)
gbrt_percentile_95 = HistGradientBoostingRegressor(
loss="quantile", quantile=0.95, max_iter=max_iter
)
gbrt_percentile_95.fit(X_train, y_train)
percentile_95_predictions = gbrt_percentile_95.predict(X_test)
现在,我们可以看看回归模型做出的预测。
last_hours = slice(-96, None)
fig, ax = plt.subplots(figsize=(15, 7))
plt.title("Predictions by regression models")
ax.plot(
y_test[last_hours],
"x-",
alpha=0.2,
label="Actual demand",
color="black",
)
ax.plot(
median_predictions[last_hours],
"^-",
label="GBRT median",
)
ax.plot(
mean_predictions[last_hours],
"x-",
label="GBRT mean (Poisson)",
)
ax.fill_between(
np.arange(96),
percentile_5_predictions[last_hours],
percentile_95_predictions[last_hours],
alpha=0.3,
label="GBRT 90% interval",
)
_ = ax.legend()
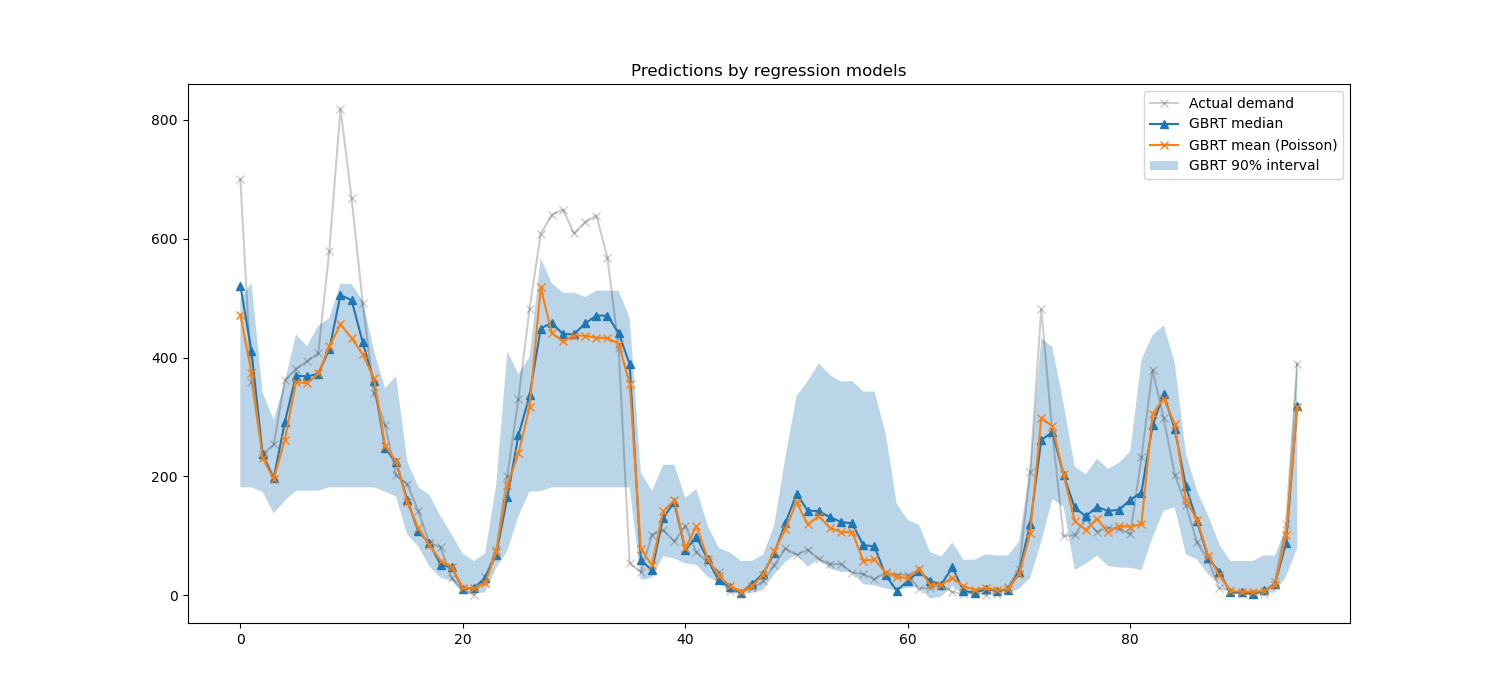
这里有趣的是,5% 和 95% 百分位数估计量之间的蓝色区域的宽度会随着一天中的时间而变化。
在夜间,蓝色带要窄得多:模型对自行车租赁数量较少相当肯定。而且,从实际需求保持在蓝色带内来看,这些预测似乎是正确的。
在白天,蓝色带要宽得多:不确定性增加,可能是因为天气的变化可能产生非常大的影响,尤其是在周末。
我们还可以看到,在工作日,通勤模式仍然体现在 5% 和 95% 的估计中。
最后,预期实际需求有 10% 的时间不在 5% 和 95% 百分位数估计之间。在这个测试区间内,实际需求似乎更高,尤其是在高峰时段。这可能表明我们的 95% 百分位数估计低估了需求峰值。这可以通过计算经验覆盖率来定量确认,如置信区间的校准中所做的那样。
查看非线性回归模型与最佳模型的性能
from sklearn.metrics import PredictionErrorDisplay
fig, axes = plt.subplots(ncols=3, figsize=(15, 6), sharey=True)
fig.suptitle("Non-linear regression models")
predictions = [
median_predictions,
percentile_5_predictions,
percentile_95_predictions,
]
labels = [
"Median",
"5th percentile",
"95th percentile",
]
for ax, pred, label in zip(axes, predictions, labels):
PredictionErrorDisplay.from_predictions(
y_true=y_test,
y_pred=pred,
kind="residual_vs_predicted",
scatter_kwargs={"alpha": 0.3},
ax=ax,
)
ax.set(xlabel="Predicted demand", ylabel="True demand")
ax.legend(["Best model", label])
plt.show()
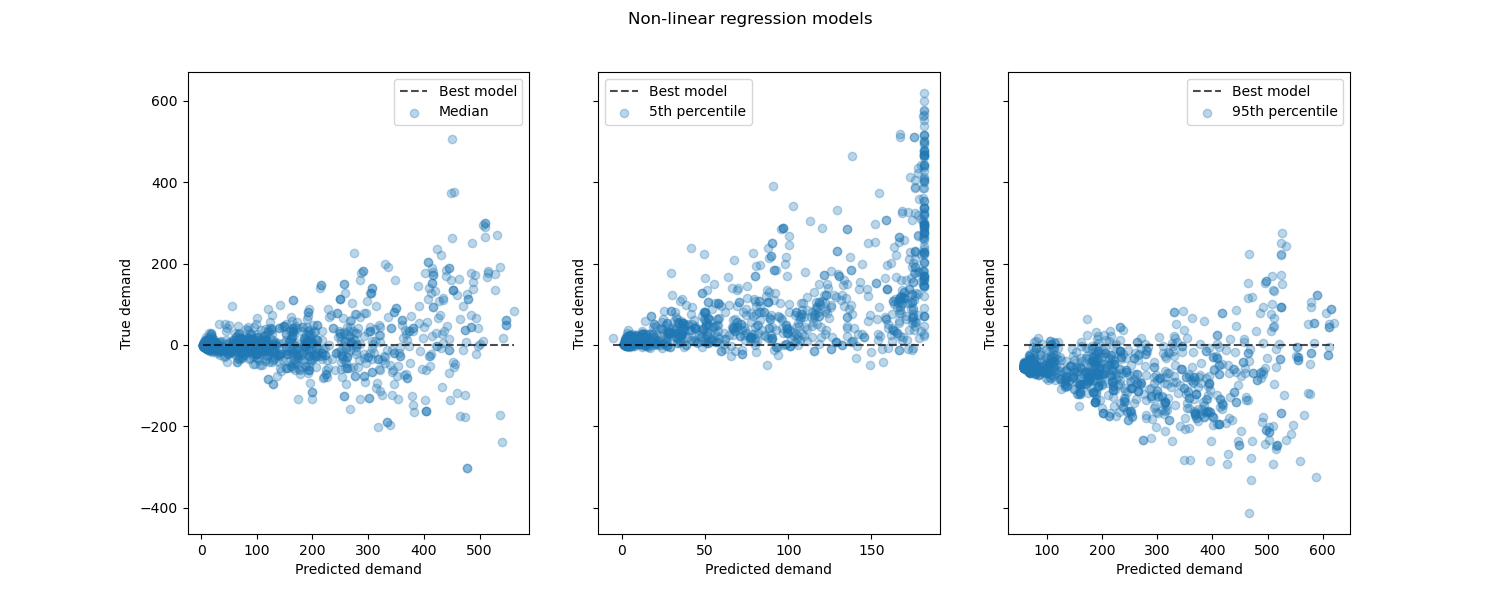
结论#
通过这个例子,我们探索了使用滞后特征的时间序列预测。我们将朴素回归(使用标准化的train_test_split
)与使用TimeSeriesSplit
的适当时间序列评估策略进行了比较。我们观察到,使用train_test_split
训练的模型(shuffle
默认为True
)产生了过于乐观的平均绝对百分比误差 (MAPE)。基于时间分割的结果更好地代表了我们时间序列回归模型的性能。我们还通过分位数回归分析了模型的预测不确定性。使用loss="quantile"
的第 5 个和第 95 个百分位数的预测为我们提供了对时间序列回归模型所做预测的不确定性的定量估计。MAPIE也可以用于不确定性估计,它提供了一种基于最近关于一致性预测方法的工作的实现,并同时估计偶然性和认知不确定性。此外,sktime 提供的功能可用于通过使用递归时间序列预测来扩展 scikit-learn 估计器,从而能够动态预测未来值。
脚本总运行时间:(0 分钟 9.974 秒)
相关示例