注意
转到末尾 下载完整示例代码,或通过 JupyterLite 或 Binder 在浏览器中运行此示例
具有多重共线性或相关特征的置换重要性#
在此示例中,我们计算了使用 威斯康星乳腺癌(诊断)数据集 训练好的 RandomForestClassifier
的特征的 置换重要性
。该模型可以在测试数据集上轻松达到约 97% 的准确率。由于此数据集包含多重共线性特征,置换重要性显示没有特征是重要的,这与高测试准确率相矛盾。
我们演示了一种处理多重共线性的可能方法,该方法包括对特征的 Spearman 秩相关系数进行层次聚类,选择一个阈值,并从每个簇中保留一个特征。
注意
# Authors: The scikit-learn developers
# SPDX-License-Identifier: BSD-3-Clause
乳腺癌数据上的随机森林特征重要性#
首先,我们定义一个函数来方便绘图
import matplotlib
from sklearn.inspection import permutation_importance
from sklearn.utils.fixes import parse_version
def plot_permutation_importance(clf, X, y, ax):
result = permutation_importance(clf, X, y, n_repeats=10, random_state=42, n_jobs=2)
perm_sorted_idx = result.importances_mean.argsort()
# `labels` argument in boxplot is deprecated in matplotlib 3.9 and has been
# renamed to `tick_labels`. The following code handles this, but as a
# scikit-learn user you probably can write simpler code by using `labels=...`
# (matplotlib < 3.9) or `tick_labels=...` (matplotlib >= 3.9).
tick_labels_parameter_name = (
"tick_labels"
if parse_version(matplotlib.__version__) >= parse_version("3.9")
else "labels"
)
tick_labels_dict = {tick_labels_parameter_name: X.columns[perm_sorted_idx]}
ax.boxplot(result.importances[perm_sorted_idx].T, vert=False, **tick_labels_dict)
ax.axvline(x=0, color="k", linestyle="--")
return ax
然后,我们在 威斯康星乳腺癌(诊断)数据集 上训练一个 RandomForestClassifier
并评估其在测试集上的准确率
from sklearn.datasets import load_breast_cancer
from sklearn.ensemble import RandomForestClassifier
from sklearn.model_selection import train_test_split
X, y = load_breast_cancer(return_X_y=True, as_frame=True)
X_train, X_test, y_train, y_test = train_test_split(X, y, random_state=42)
clf = RandomForestClassifier(n_estimators=100, random_state=42)
clf.fit(X_train, y_train)
print(f"Baseline accuracy on test data: {clf.score(X_test, y_test):.2}")
Baseline accuracy on test data: 0.97
接下来,我们绘制基于树的特征重要性和置换重要性。置换重要性是在训练集上计算的,以显示模型在训练过程中对每个特征的依赖程度。
import matplotlib.pyplot as plt
import numpy as np
import pandas as pd
mdi_importances = pd.Series(clf.feature_importances_, index=X_train.columns)
tree_importance_sorted_idx = np.argsort(clf.feature_importances_)
fig, (ax1, ax2) = plt.subplots(1, 2, figsize=(12, 8))
mdi_importances.sort_values().plot.barh(ax=ax1)
ax1.set_xlabel("Gini importance")
plot_permutation_importance(clf, X_train, y_train, ax2)
ax2.set_xlabel("Decrease in accuracy score")
fig.suptitle(
"Impurity-based vs. permutation importances on multicollinear features (train set)"
)
_ = fig.tight_layout()
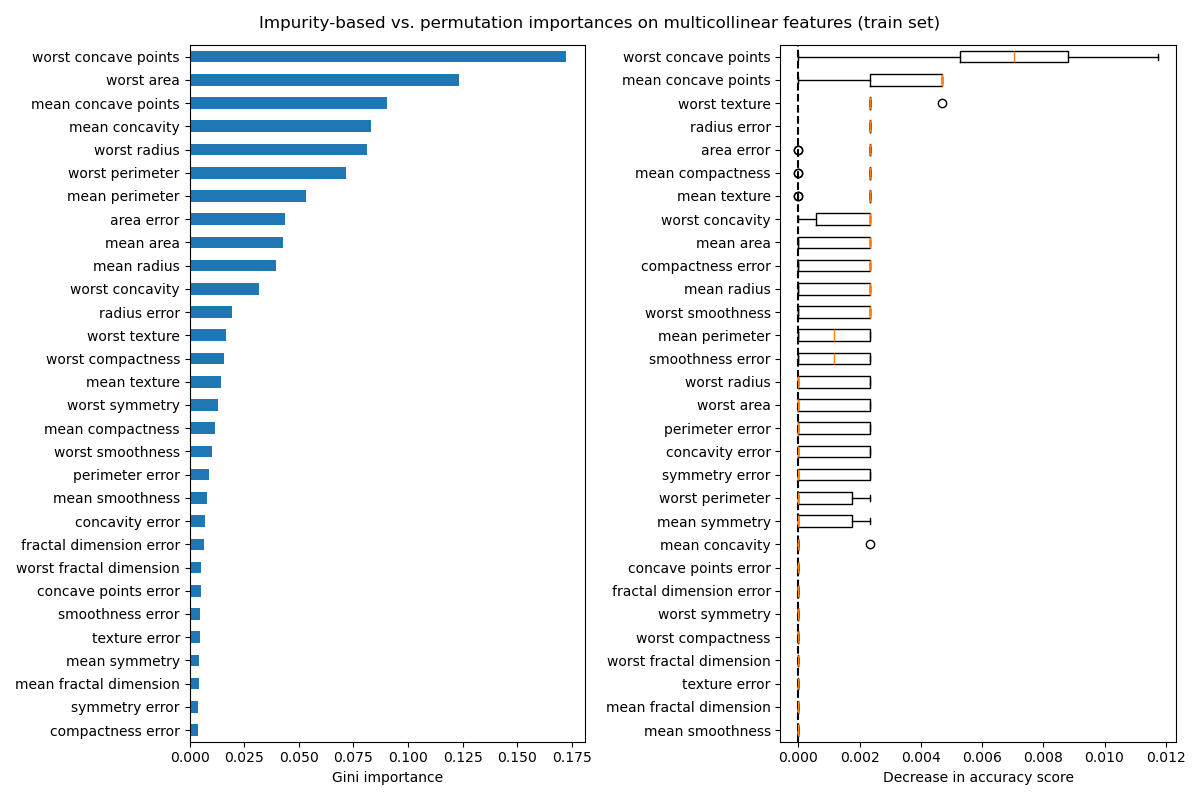
左侧图显示了模型的 Gini 重要性。由于 scikit-learn 的 RandomForestClassifier
实现使用随机子集 \(\sqrt{n_\text{features}}\) 特征在每次分裂时,它能够稀释任何单个相关特征的优势。因此,单个特征的重要性可能在相关特征之间分布得更均匀。由于特征基数大且分类器未过拟合,我们可以相对信任这些值。
右侧图中的置换重要性显示,置换一个特征最多使准确率下降 0.012
,这表明没有特征是重要的。这与作为基线计算的高测试准确率相矛盾:某些特征必然是重要的。
同样,在测试集上计算的准确率得分变化似乎是偶然的
fig, ax = plt.subplots(figsize=(7, 6))
plot_permutation_importance(clf, X_test, y_test, ax)
ax.set_title("Permutation Importances on multicollinear features\n(test set)")
ax.set_xlabel("Decrease in accuracy score")
_ = ax.figure.tight_layout()
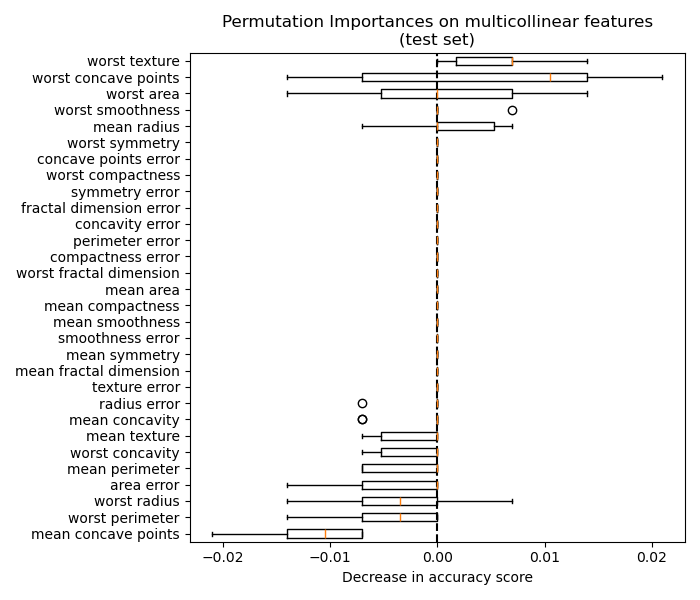
然而,在存在相关特征的情况下,仍然可以计算有意义的置换重要性,如下一节所示。
处理多重共线性特征#
当特征共线时,置换一个特征对模型性能影响很小,因为它可以从相关特征中获取相同的信息。请注意,并非所有预测模型都是如此,这取决于它们的底层实现。
处理多重共线性特征的一种方法是对 Spearman 秩相关系数进行层次聚类,选择一个阈值,并从每个簇中保留一个特征。首先,我们绘制相关特征的热图
from scipy.cluster import hierarchy
from scipy.spatial.distance import squareform
from scipy.stats import spearmanr
fig, (ax1, ax2) = plt.subplots(1, 2, figsize=(12, 8))
corr = spearmanr(X).correlation
# Ensure the correlation matrix is symmetric
corr = (corr + corr.T) / 2
np.fill_diagonal(corr, 1)
# We convert the correlation matrix to a distance matrix before performing
# hierarchical clustering using Ward's linkage.
distance_matrix = 1 - np.abs(corr)
dist_linkage = hierarchy.ward(squareform(distance_matrix))
dendro = hierarchy.dendrogram(
dist_linkage, labels=X.columns.to_list(), ax=ax1, leaf_rotation=90
)
dendro_idx = np.arange(0, len(dendro["ivl"]))
ax2.imshow(corr[dendro["leaves"], :][:, dendro["leaves"]])
ax2.set_xticks(dendro_idx)
ax2.set_yticks(dendro_idx)
ax2.set_xticklabels(dendro["ivl"], rotation="vertical")
ax2.set_yticklabels(dendro["ivl"])
_ = fig.tight_layout()
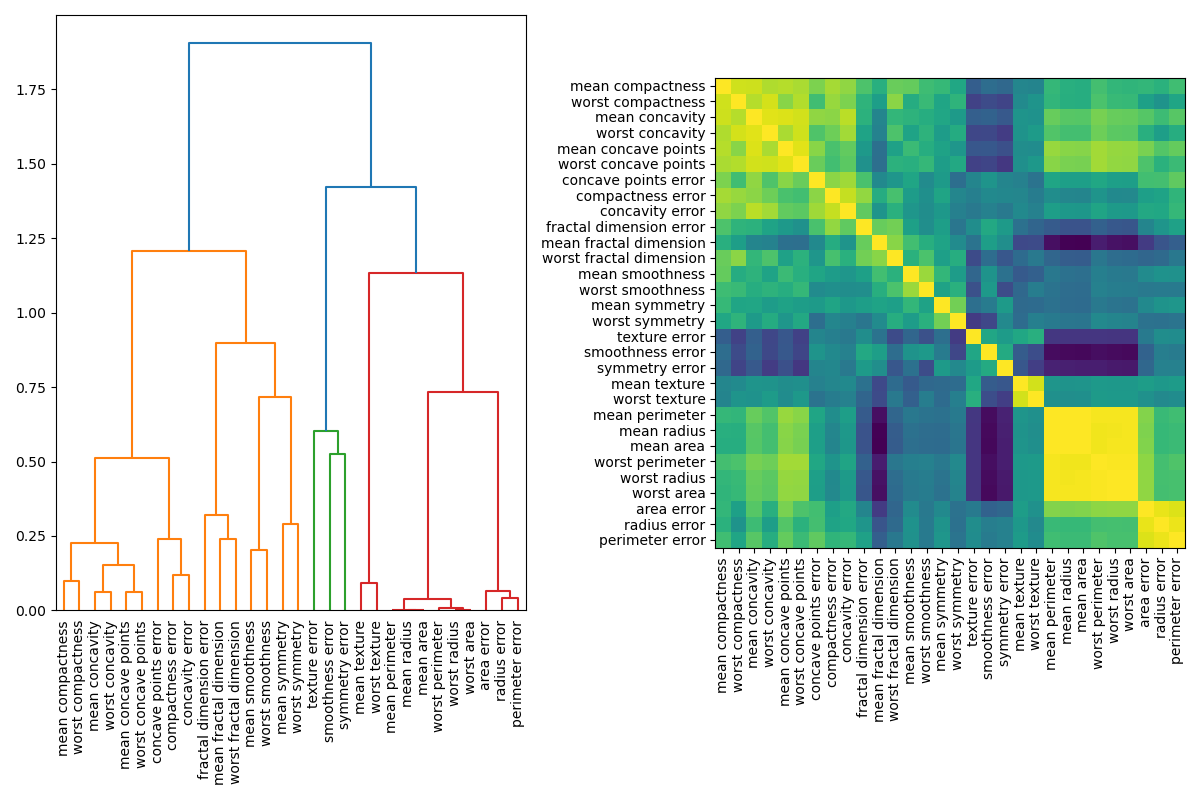
接下来,我们通过目视检查树状图手动选择一个阈值,将我们的特征分组到簇中,并从每个簇中选择一个特征进行保留,从我们的数据集中选择这些特征,然后训练一个新的随机森林。与在完整数据集上训练的随机森林相比,新随机森林的测试准确率没有太大变化。
from collections import defaultdict
cluster_ids = hierarchy.fcluster(dist_linkage, 1, criterion="distance")
cluster_id_to_feature_ids = defaultdict(list)
for idx, cluster_id in enumerate(cluster_ids):
cluster_id_to_feature_ids[cluster_id].append(idx)
selected_features = [v[0] for v in cluster_id_to_feature_ids.values()]
selected_features_names = X.columns[selected_features]
X_train_sel = X_train[selected_features_names]
X_test_sel = X_test[selected_features_names]
clf_sel = RandomForestClassifier(n_estimators=100, random_state=42)
clf_sel.fit(X_train_sel, y_train)
print(
"Baseline accuracy on test data with features removed:"
f" {clf_sel.score(X_test_sel, y_test):.2}"
)
Baseline accuracy on test data with features removed: 0.97
我们最终可以探索所选特征子集的置换重要性
fig, ax = plt.subplots(figsize=(7, 6))
plot_permutation_importance(clf_sel, X_test_sel, y_test, ax)
ax.set_title("Permutation Importances on selected subset of features\n(test set)")
ax.set_xlabel("Decrease in accuracy score")
ax.figure.tight_layout()
plt.show()
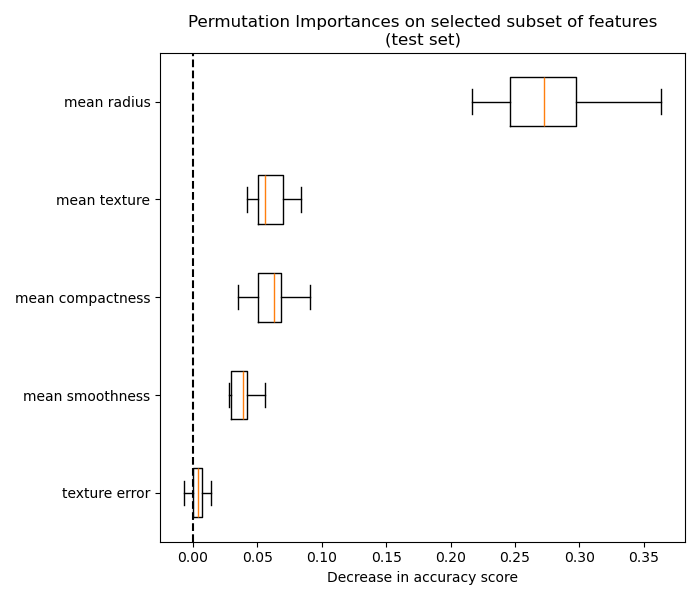
脚本总运行时间: (0 分钟 4.272 秒)
相关示例