注意
转到末尾 下载完整的示例代码。或通过 JupyterLite 或 Binder 在您的浏览器中运行此示例
随机森林的袋外误差#
RandomForestClassifier
使用 *自助聚集* 进行训练,其中每棵新树都根据训练观测值的自助样本 \(z_i = (x_i, y_i)\) 进行拟合。 *袋外* (OOB) 误差是每个 \(z_i\) 的平均误差,使用不包含 \(z_i\) 的树的预测来计算。这允许在训练 RandomForestClassifier
的同时进行拟合和验证[1]。
下面的示例演示了如何在训练期间每添加一棵新树时测量 OOB 误差。生成的图表允许从业人员估算 n_estimators
的合适值,在此值下误差趋于稳定。
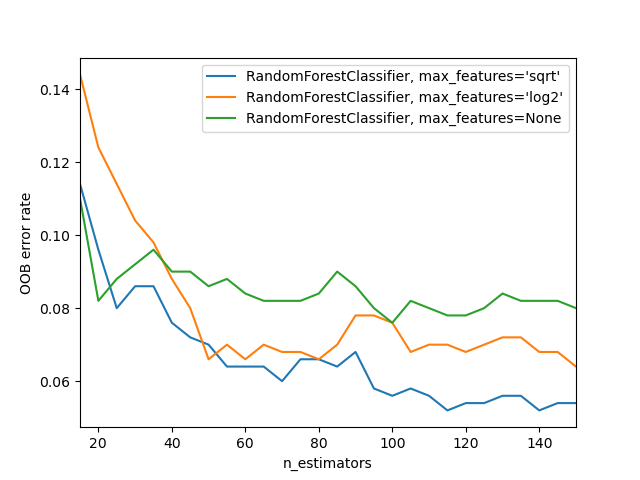
# Authors: The scikit-learn developers
# SPDX-License-Identifier: BSD-3-Clause
from collections import OrderedDict
import matplotlib.pyplot as plt
from sklearn.datasets import make_classification
from sklearn.ensemble import RandomForestClassifier
RANDOM_STATE = 123
# Generate a binary classification dataset.
X, y = make_classification(
n_samples=500,
n_features=25,
n_clusters_per_class=1,
n_informative=15,
random_state=RANDOM_STATE,
)
# NOTE: Setting the `warm_start` construction parameter to `True` disables
# support for parallelized ensembles but is necessary for tracking the OOB
# error trajectory during training.
ensemble_clfs = [
(
"RandomForestClassifier, max_features='sqrt'",
RandomForestClassifier(
warm_start=True,
oob_score=True,
max_features="sqrt",
random_state=RANDOM_STATE,
),
),
(
"RandomForestClassifier, max_features='log2'",
RandomForestClassifier(
warm_start=True,
max_features="log2",
oob_score=True,
random_state=RANDOM_STATE,
),
),
(
"RandomForestClassifier, max_features=None",
RandomForestClassifier(
warm_start=True,
max_features=None,
oob_score=True,
random_state=RANDOM_STATE,
),
),
]
# Map a classifier name to a list of (<n_estimators>, <error rate>) pairs.
error_rate = OrderedDict((label, []) for label, _ in ensemble_clfs)
# Range of `n_estimators` values to explore.
min_estimators = 15
max_estimators = 150
for label, clf in ensemble_clfs:
for i in range(min_estimators, max_estimators + 1, 5):
clf.set_params(n_estimators=i)
clf.fit(X, y)
# Record the OOB error for each `n_estimators=i` setting.
oob_error = 1 - clf.oob_score_
error_rate[label].append((i, oob_error))
# Generate the "OOB error rate" vs. "n_estimators" plot.
for label, clf_err in error_rate.items():
xs, ys = zip(*clf_err)
plt.plot(xs, ys, label=label)
plt.xlim(min_estimators, max_estimators)
plt.xlabel("n_estimators")
plt.ylabel("OOB error rate")
plt.legend(loc="upper right")
plt.show()
脚本总运行时间:(0 分钟 4.478 秒)
相关示例