注意
转到结尾 下载完整的示例代码。或者通过 JupyterLite 或 Binder 在浏览器中运行此示例。
均值漂移聚类算法演示#
参考文献
Dorin Comaniciu 和 Peter Meer,“均值漂移:一种稳健的特征空间分析方法”。IEEE 模式分析与机器智能汇刊。2002 年。第 603-619 页。
# Authors: The scikit-learn developers
# SPDX-License-Identifier: BSD-3-Clause
import numpy as np
from sklearn.cluster import MeanShift, estimate_bandwidth
from sklearn.datasets import make_blobs
生成样本数据#
centers = [[1, 1], [-1, -1], [1, -1]]
X, _ = make_blobs(n_samples=10000, centers=centers, cluster_std=0.6)
使用 MeanShift 计算聚类#
# The following bandwidth can be automatically detected using
bandwidth = estimate_bandwidth(X, quantile=0.2, n_samples=500)
ms = MeanShift(bandwidth=bandwidth, bin_seeding=True)
ms.fit(X)
labels = ms.labels_
cluster_centers = ms.cluster_centers_
labels_unique = np.unique(labels)
n_clusters_ = len(labels_unique)
print("number of estimated clusters : %d" % n_clusters_)
number of estimated clusters : 3
绘制结果#
import matplotlib.pyplot as plt
plt.figure(1)
plt.clf()
colors = ["#dede00", "#377eb8", "#f781bf"]
markers = ["x", "o", "^"]
for k, col in zip(range(n_clusters_), colors):
my_members = labels == k
cluster_center = cluster_centers[k]
plt.plot(X[my_members, 0], X[my_members, 1], markers[k], color=col)
plt.plot(
cluster_center[0],
cluster_center[1],
markers[k],
markerfacecolor=col,
markeredgecolor="k",
markersize=14,
)
plt.title("Estimated number of clusters: %d" % n_clusters_)
plt.show()
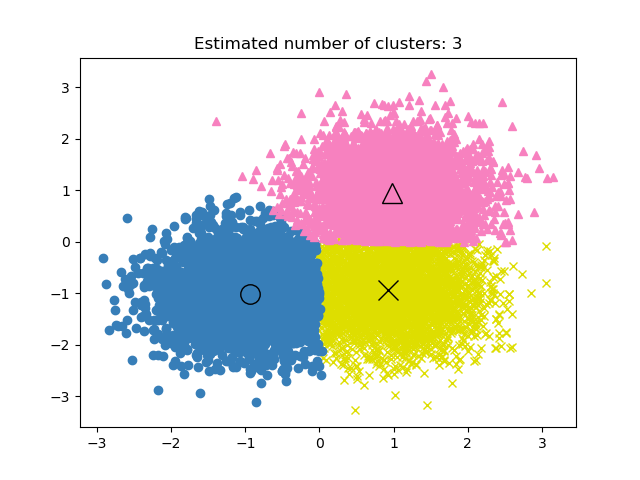
脚本总运行时间:(0 分钟 0.441 秒)
相关示例