注意
转到结尾 下载完整的示例代码。或者通过 JupyterLite 或 Binder 在您的浏览器中运行此示例
DBSCAN 聚类算法演示#
DBSCAN(基于密度的噪声应用空间聚类)在高密度区域查找核心样本,并从中扩展聚类。此算法适用于包含相似密度聚类的数据。
请参阅玩具数据集上不同聚类算法的比较示例,了解不同聚类算法在二维数据集上的演示。
# Authors: The scikit-learn developers
# SPDX-License-Identifier: BSD-3-Clause
数据生成#
我们使用make_blobs
创建3个合成聚类。
from sklearn.datasets import make_blobs
from sklearn.preprocessing import StandardScaler
centers = [[1, 1], [-1, -1], [1, -1]]
X, labels_true = make_blobs(
n_samples=750, centers=centers, cluster_std=0.4, random_state=0
)
X = StandardScaler().fit_transform(X)
我们可以可视化生成的数据
import matplotlib.pyplot as plt
plt.scatter(X[:, 0], X[:, 1])
plt.show()
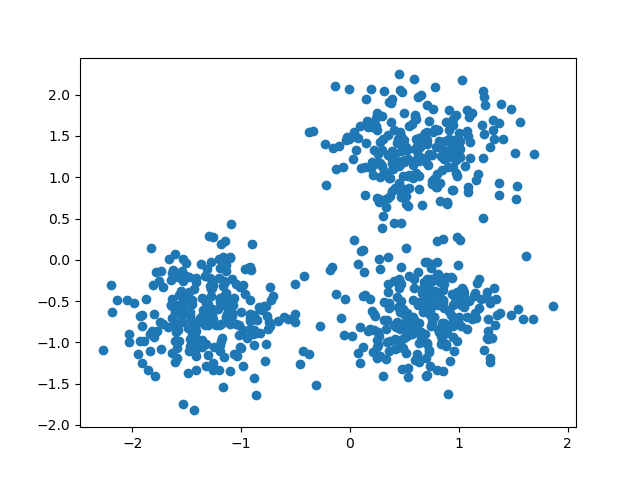
计算DBSCAN#
可以使用DBSCAN
的labels_
属性访问其分配的标签。噪声样本的标签为math:-1
。
import numpy as np
from sklearn import metrics
from sklearn.cluster import DBSCAN
db = DBSCAN(eps=0.3, min_samples=10).fit(X)
labels = db.labels_
# Number of clusters in labels, ignoring noise if present.
n_clusters_ = len(set(labels)) - (1 if -1 in labels else 0)
n_noise_ = list(labels).count(-1)
print("Estimated number of clusters: %d" % n_clusters_)
print("Estimated number of noise points: %d" % n_noise_)
Estimated number of clusters: 3
Estimated number of noise points: 18
聚类算法从根本上说是无监督学习方法。但是,由于make_blobs
可以访问合成聚类的真实标签,因此可以使用利用此“监督”地面真实信息的评估指标来量化结果聚类的质量。此类指标的示例包括同质性、完整性、V-测度、Rand指数、调整后的Rand指数和调整后的互信息 (AMI)。
如果不知道地面真实标签,则只能使用模型结果本身进行评估。在这种情况下,轮廓系数非常有用。
更多信息,请参阅聚类性能评估中的偶然性调整示例或聚类性能评估模块。
print(f"Homogeneity: {metrics.homogeneity_score(labels_true, labels):.3f}")
print(f"Completeness: {metrics.completeness_score(labels_true, labels):.3f}")
print(f"V-measure: {metrics.v_measure_score(labels_true, labels):.3f}")
print(f"Adjusted Rand Index: {metrics.adjusted_rand_score(labels_true, labels):.3f}")
print(
"Adjusted Mutual Information:"
f" {metrics.adjusted_mutual_info_score(labels_true, labels):.3f}"
)
print(f"Silhouette Coefficient: {metrics.silhouette_score(X, labels):.3f}")
Homogeneity: 0.953
Completeness: 0.883
V-measure: 0.917
Adjusted Rand Index: 0.952
Adjusted Mutual Information: 0.916
Silhouette Coefficient: 0.626
结果图#
核心样本(大点)和非核心样本(小点)根据分配的聚类进行颜色编码。标记为噪声的样本以黑色表示。
unique_labels = set(labels)
core_samples_mask = np.zeros_like(labels, dtype=bool)
core_samples_mask[db.core_sample_indices_] = True
colors = [plt.cm.Spectral(each) for each in np.linspace(0, 1, len(unique_labels))]
for k, col in zip(unique_labels, colors):
if k == -1:
# Black used for noise.
col = [0, 0, 0, 1]
class_member_mask = labels == k
xy = X[class_member_mask & core_samples_mask]
plt.plot(
xy[:, 0],
xy[:, 1],
"o",
markerfacecolor=tuple(col),
markeredgecolor="k",
markersize=14,
)
xy = X[class_member_mask & ~core_samples_mask]
plt.plot(
xy[:, 0],
xy[:, 1],
"o",
markerfacecolor=tuple(col),
markeredgecolor="k",
markersize=6,
)
plt.title(f"Estimated number of clusters: {n_clusters_}")
plt.show()
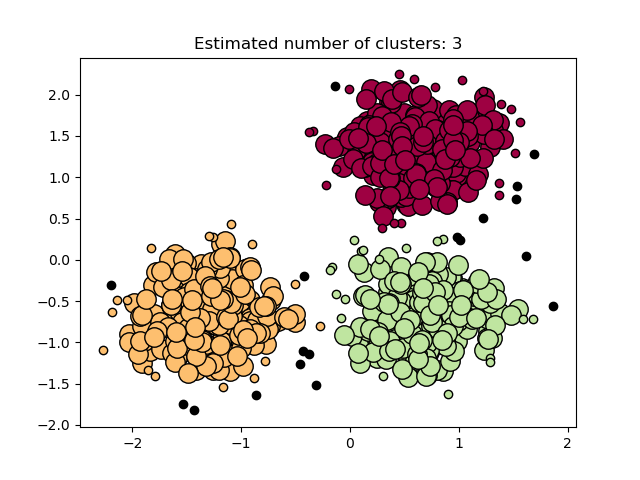
脚本总运行时间:(0分钟0.189秒)
相关示例