比较随机森林和多输出元估计器#
一个示例,用于比较随机森林的多输出回归和 multioutput.MultiOutputRegressor 元估计器。
此示例说明了如何使用 multioutput.MultiOutputRegressor 元估计器执行多输出回归。使用随机森林回归器,它本身支持多输出回归,因此可以比较结果。
随机森林回归器只会预测每个目标的观测值范围内或更接近零的值。因此,预测偏向于圆心。
使用单个基础特征,模型学习 x 和 y 坐标作为输出。
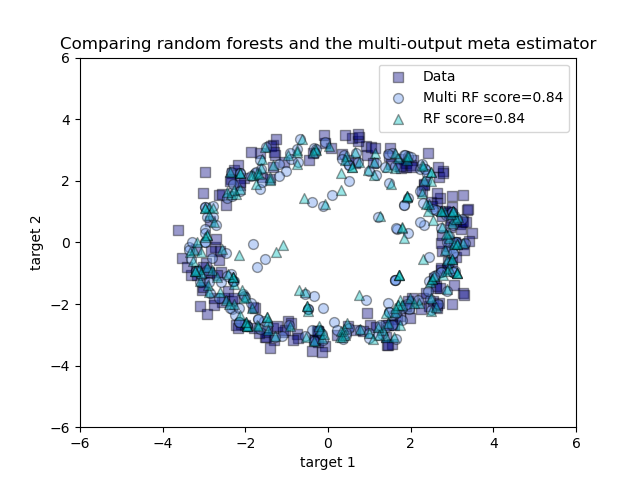
# Author: Tim Head <[email protected]>
#
# License: BSD 3 clause
import matplotlib.pyplot as plt
import numpy as np
from sklearn.ensemble import RandomForestRegressor
from sklearn.model_selection import train_test_split
from sklearn.multioutput import MultiOutputRegressor
# Create a random dataset
rng = np.random.RandomState(1)
X = np.sort(200 * rng.rand(600, 1) - 100, axis=0)
y = np.array([np.pi * np.sin(X).ravel(), np.pi * np.cos(X).ravel()]).T
y += 0.5 - rng.rand(*y.shape)
X_train, X_test, y_train, y_test = train_test_split(
X, y, train_size=400, test_size=200, random_state=4
)
max_depth = 30
regr_multirf = MultiOutputRegressor(
RandomForestRegressor(n_estimators=100, max_depth=max_depth, random_state=0)
)
regr_multirf.fit(X_train, y_train)
regr_rf = RandomForestRegressor(n_estimators=100, max_depth=max_depth, random_state=2)
regr_rf.fit(X_train, y_train)
# Predict on new data
y_multirf = regr_multirf.predict(X_test)
y_rf = regr_rf.predict(X_test)
# Plot the results
plt.figure()
s = 50
a = 0.4
plt.scatter(
y_test[:, 0],
y_test[:, 1],
edgecolor="k",
c="navy",
s=s,
marker="s",
alpha=a,
label="Data",
)
plt.scatter(
y_multirf[:, 0],
y_multirf[:, 1],
edgecolor="k",
c="cornflowerblue",
s=s,
alpha=a,
label="Multi RF score=%.2f" % regr_multirf.score(X_test, y_test),
)
plt.scatter(
y_rf[:, 0],
y_rf[:, 1],
edgecolor="k",
c="c",
s=s,
marker="^",
alpha=a,
label="RF score=%.2f" % regr_rf.score(X_test, y_test),
)
plt.xlim([-6, 6])
plt.ylim([-6, 6])
plt.xlabel("target 1")
plt.ylabel("target 2")
plt.title("Comparing random forests and the multi-output meta estimator")
plt.legend()
plt.show()
脚本总运行时间:(0 分钟 0.520 秒)
相关示例