注意
转到结尾 下载完整的示例代码。或者通过 JupyterLite 或 Binder 在您的浏览器中运行此示例
通过信息准则进行 Lasso 模型选择#
此示例重现了 [ZHT2007] 图 2 的示例。一个 LassoLarsIC
估计器拟合到糖尿病数据集上,并使用 AIC 和 BIC 准则来选择最佳模型。
注意
需要注意的是,使用 LassoLarsIC
查找 alpha
的优化依赖于样本内计算的 AIC 或 BIC 准则,因此直接依赖于训练集。这种方法不同于交叉验证程序。有关这两种方法的比较,您可以参考以下示例:Lasso 模型选择:AIC-BIC / 交叉验证。
参考文献
# Authors: The scikit-learn developers
# SPDX-License-Identifier: BSD-3-Clause
我们将使用糖尿病数据集。
from sklearn.datasets import load_diabetes
X, y = load_diabetes(return_X_y=True, as_frame=True)
n_samples = X.shape[0]
X.head()
Scikit-learn 提供了一个名为 LassoLarsIC
的估计器,它使用 Akaike 信息准则 (AIC) 或贝叶斯信息准则 (BIC) 来选择最佳模型。在拟合此模型之前,我们将缩放数据集。
在下文中,我们将拟合两个模型来比较 AIC 和 BIC 报告的值。
from sklearn.linear_model import LassoLarsIC
from sklearn.pipeline import make_pipeline
from sklearn.preprocessing import StandardScaler
lasso_lars_ic = make_pipeline(StandardScaler(), LassoLarsIC(criterion="aic")).fit(X, y)
为了与 [ZHT2007] 中的定义一致,我们需要重新缩放 AIC 和 BIC。事实上,与从线性模型的最大对数似然推导出的 AIC 的原始定义相比,Zou 等人忽略了一些常数项。您可以参考 用户指南的数学细节部分。
import numpy as np
aic_criterion = zou_et_al_criterion_rescaling(
lasso_lars_ic[-1].criterion_,
n_samples,
lasso_lars_ic[-1].noise_variance_,
)
index_alpha_path_aic = np.flatnonzero(
lasso_lars_ic[-1].alphas_ == lasso_lars_ic[-1].alpha_
)[0]
lasso_lars_ic.set_params(lassolarsic__criterion="bic").fit(X, y)
bic_criterion = zou_et_al_criterion_rescaling(
lasso_lars_ic[-1].criterion_,
n_samples,
lasso_lars_ic[-1].noise_variance_,
)
index_alpha_path_bic = np.flatnonzero(
lasso_lars_ic[-1].alphas_ == lasso_lars_ic[-1].alpha_
)[0]
现在我们已经收集了 AIC 和 BIC,我们也可以检查这两个准则的最小值是否发生在相同的 alpha 值。然后,我们可以简化下面的图。
index_alpha_path_aic == index_alpha_path_bic
np.True_
最后,我们可以绘制 AIC 和 BIC 准则以及随后选择的正则化参数。
import matplotlib.pyplot as plt
plt.plot(aic_criterion, color="tab:blue", marker="o", label="AIC criterion")
plt.plot(bic_criterion, color="tab:orange", marker="o", label="BIC criterion")
plt.vlines(
index_alpha_path_bic,
aic_criterion.min(),
aic_criterion.max(),
color="black",
linestyle="--",
label="Selected alpha",
)
plt.legend()
plt.ylabel("Information criterion")
plt.xlabel("Lasso model sequence")
_ = plt.title("Lasso model selection via AIC and BIC")
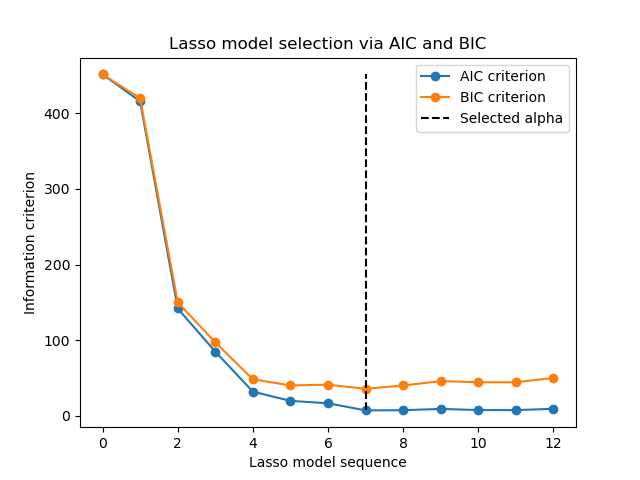
脚本总运行时间:(0 分 0.107 秒)
相关示例